Understanding the Role of Data Analytics in Strategic Decision-Making
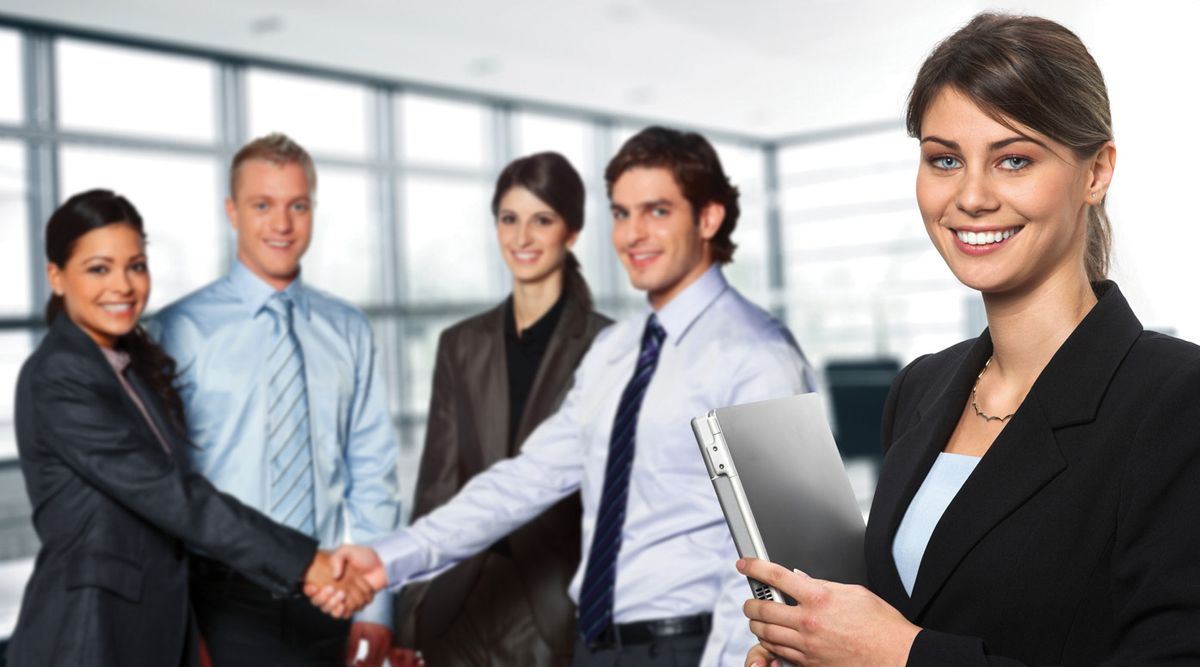
Enhancing Business Decisions with Data
In the realm of business intelligence, data analytics serves as a compass for navigating the complex landscape of strategic choices. It empowers organizations to move beyond intuition, providing a foundation of evidence upon which to base critical decisions. By harnessing the power of data, companies can uncover trends, optimize pricing, and enhance customer satisfaction, ensuring that each decision is informed by concrete insights rather than conjecture.
Data-driven decision-making is pivotal for businesses seeking to improve their accuracy and efficiency. The insights gleaned from data analysis enable organizations to identify patterns and insights that lead to smarter, more strategic choices. This approach not only reduces the risk of errors but also helps in allocating resources more effectively, paving the way for growth and increased competitiveness.
The integration of data analytics into business processes transcends traditional boundaries, affecting various aspects of operations. From supply chain optimization to personalized marketing and product innovation, data analysis offers a comprehensive view that can revolutionize business practices.
Embracing data analytics is synonymous with embracing a future of informed, bold steps. It is a critical component for any business that aims to remain competitive in a data-driven world.
Risks and Challenges in Data-Driven Decision Making
While data-driven decision making (DDDM) can significantly enhance strategic choices, it is not without its challenges. Managing data quality and integrity is a primary concern, as poor data can lead to misguided decisions. Overcoming data silos within an organization is also crucial to ensure that information is shared and utilized effectively.
Bridging the skills gap is essential, as a team proficient in data analysis is necessary to extract meaningful insights. Keeping up with rapidly evolving technology and ensuring data privacy and security are further challenges that require ongoing attention and resources.
To effectively overcome these challenges, businesses must focus on key performance indicators (KPIs) and invest in the right tools and training. Harnessing predictive analytics can be particularly beneficial for future planning, as it allows organizations to anticipate trends and consumer behavior. The following list outlines strategic solutions to address these hurdles:
- Establishing strong data governance
- Promoting data integration across departments
- Investing in continuous training for data literacy
- Staying updated with the latest technology
- Developing robust data privacy strategies
- Focusing on actionable insights from KPIs
Case Study: Netflix's Data Utilization
Netflix's ascent to the pinnacle of the streaming industry is a testament to the power of data analytics in strategic decision-making. By meticulously analyzing user data, Netflix has crafted a highly personalized experience for its viewers. The recommendation algorithm stands out, utilizing viewing history, ratings, and time-of-day patterns to suggest content that resonates with individual preferences.
Personalization is not the only benefit; data analytics has also guided Netflix in developing original content that captivates audiences, contributing to its market dominance. The table below highlights the impact of data utilization on Netflix's performance:
Aspect | Impact of Data Analytics |
---|---|
Content Personalization | Enhanced user engagement and satisfaction |
Original Content Development | Increased viewership and subscriber growth |
Service Optimization | Improved streaming quality and user experience |
Embracing a data-driven approach has enabled Netflix to not only meet but often exceed viewer expectations, setting a new standard for the industry.
The success story of Netflix underscores the significance of leveraging analytics to inform content strategy and operational improvements. It serves as a compelling example for businesses aiming to harness the potential of data to drive smarter decisions.
Measuring the Impact of Data Analytics on Business Performance
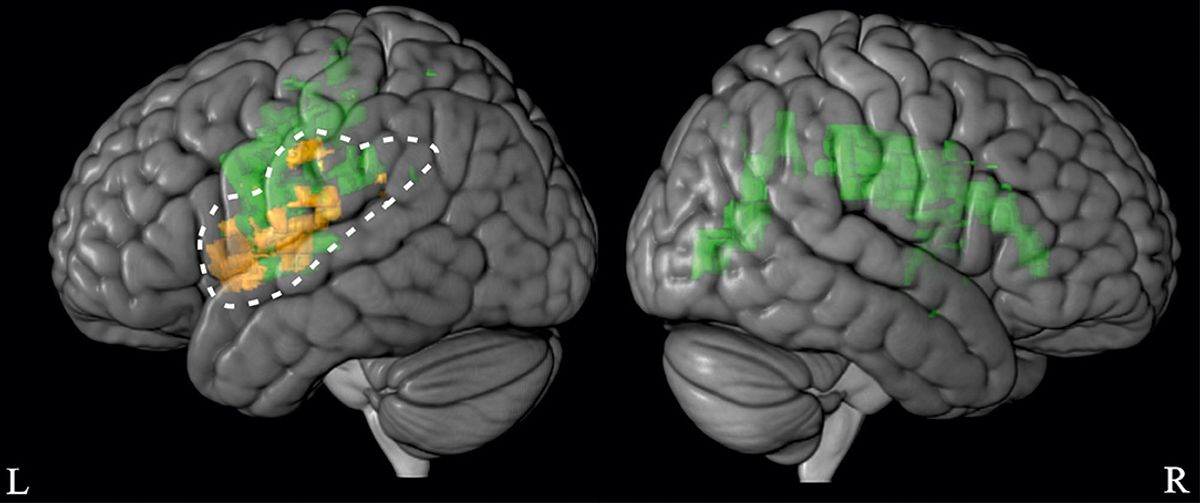
Key Performance Indicators for Data Analytics
To gauge the effectiveness of data analytics within an organization, it's essential to track Key Performance Indicators (KPIs). These metrics provide a clear view of how data-driven strategies are influencing various aspects of business performance. Selecting the right KPIs is crucial; they should align with strategic goals and be tailored to the specific needs of the business.
Here are some fundamental KPIs to consider:
- Return on Investment (ROI): Measure the financial returns compared to the cost of your data analytics initiatives.
- Customer Satisfaction: Monitor feedback to gauge the effectiveness of data-driven decisions on customer experience.
- Operational Efficiency: Track improvements in processes, such as reduced costs or increased productivity.
- Market Share: Analyze shifts in market share to assess the competitive impact of your strategies.
It's not just about having data; it's about turning that data into actionable insights that drive business growth. Regularly reviewing and adapting your approach to KPIs ensures ongoing relevance and impact.
Interpreting Data Analytics Results
Interpreting data analytics results is crucial for transforming complex data findings into understandable insights that drive strategic decision-making. It's essential to align these insights with the predefined business objectives to ensure they are relevant and actionable.
- Return on Investment (ROI): Measure the financial impact of your data analytics initiatives.
- Customer Satisfaction: Monitor to gauge the effectiveness of your data-driven decisions.
- Operational Efficiency: Track improvements such as reduced costs or increased productivity.
- Market Share: Analyze changes in market share to understand the impact of your data-driven strategies.
By comparing results against goals and considering the business context, organizations can draw meaningful conclusions that enhance performance and growth. It is also important to be aware of limitations, assumptions, and potential biases that could affect the quality of the decisions made.
In practice, data-driven leadership not only informs strategy but also enhances employee engagement, retention, and recruitment. The ability to translate data into actionable insights is key for success in today's competitive landscape.
Benchmarking Against Industry Standards
Benchmarking against industry standards is a critical step in ensuring that your analytics practices are competitive and effective. Consistency and timeliness are key in monitoring and evaluating data against set standards. To achieve this, businesses should:
- Implement Automated Data Monitoring Tools to automatically monitor data for errors, anomalies, or inconsistencies, alerting teams to issues in real time.
- Schedule Regular Data Cleansing to remove or correct inaccuracies, duplicates, and irrelevant information, keeping the data sets current and useful.
- Establish standardized data collection processes across various channels and touchpoints to minimize inconsistencies and errors, enhancing data integrity.
- Leverage data integration tools like Improvado to automate the process of aggregating data from multiple sources, standardizing datasets, and ensuring that data is consistent.
It is essential to stay agile, continuously updating data and analysis methods to reflect current market trends, emerging technologies, and changing customer behaviors. This agility supports the integration of analytics for business growth, prioritizes data governance for quality insights, and harnesses real-time data for agile decision-making.
By comparing your analytics outcomes with those of your peers, you can identify areas for improvement and opportunities to innovate. Remember, the goal is not just to match but to exceed industry benchmarks, thereby gaining a competitive edge. Data governance plays a pivotal role in this process, ensuring the quality and reliability of the insights you derive from your analytics efforts.
Advanced Analytics Techniques for Predictive Insights
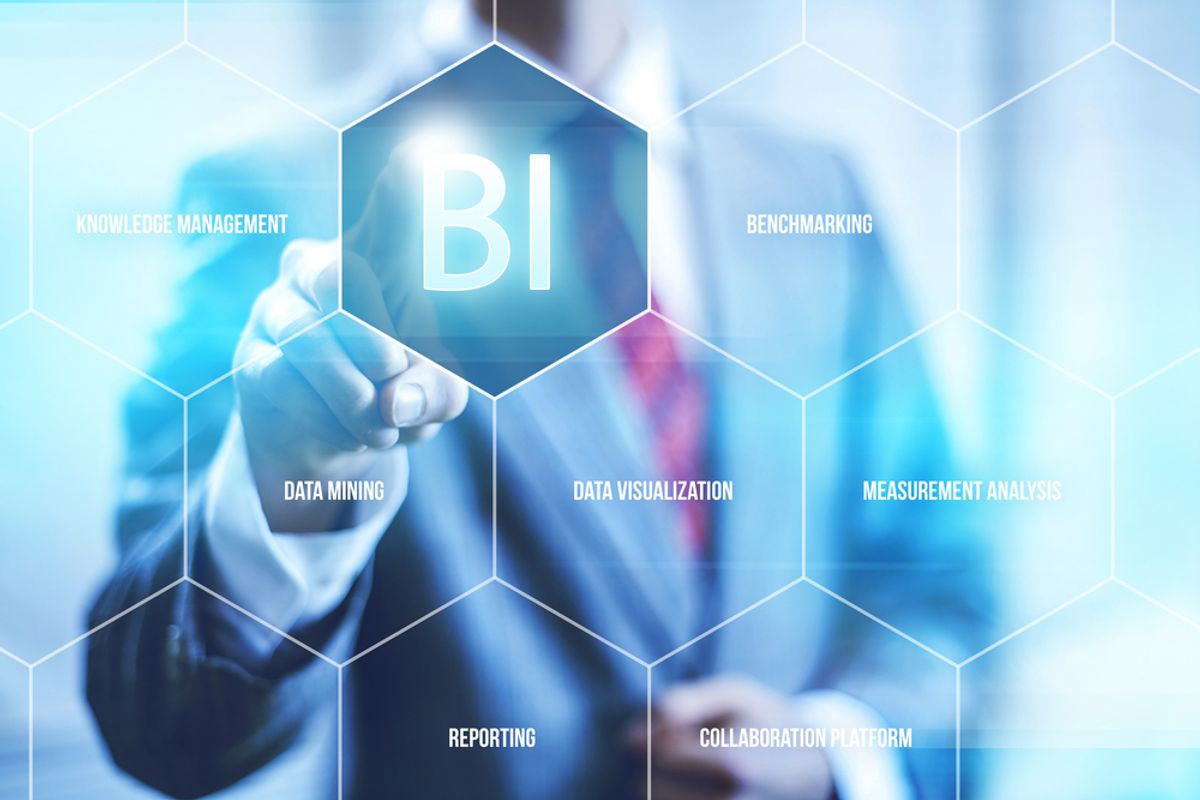
Predictive vs. Prescriptive Analytics
Understanding the distinction between predictive and prescriptive analytics is crucial for businesses aiming to leverage data for strategic advantage. Predictive analytics harnesses historical data to forecast future events, answering questions like "What's likely to happen?" This approach is invaluable for anticipating market trends, customer behaviors, and potential risks.
On the other hand, prescriptive analytics recommends specific actions to achieve desired outcomes. It addresses the question of "What should we do?" by employing techniques such as optimization and simulation. This type of analytics can guide businesses to make data-informed decisions swiftly, enhancing operational efficiency and performance.
By integrating both predictive and prescriptive analytics, organizations can not only foresee future challenges but also devise and implement the best strategies to address them.
Here's a comparison of how each type of analytics contributes to decision-making:
- Predictive Analytics: Forecasts future trends using machine learning and statistical modeling.
- Prescriptive Analytics: Suggests actionable strategies and improvements, like optimal pricing or supply chain enhancements.
Forecasting Customer Demand
Demand forecasting is a critical component of strategic business planning. It involves the use of predictive analytics to analyze historical data and identify patterns that can inform future business decisions. Accurate demand forecasting enables companies to optimize inventory levels, ensuring they meet customer needs without overstocking.
By leveraging data from past sales, market trends, and consumer behaviors, businesses gain the ability to anticipate market demands and adjust their strategies accordingly.
The process of forecasting is not just about numbers; it's about understanding customer insights and translating them into actionable business intelligence. Here's a simplified breakdown of the steps involved in demand forecasting:
- Collecting and cleaning historical sales data.
- Analyzing market conditions and consumer behavior.
- Incorporating external data such as industry trends and economic indicators.
- Applying statistical models to predict future demand.
- Continuously refining the models with new data and insights.
Optimizing Pricing Strategies
In the realm of analytics, dynamic pricing stands out as a pivotal strategy for maximizing revenue. Businesses can adjust prices in real-time based on various factors such as demand, seasonality, and consumer behavior. This approach not only enhances competitiveness but also ensures a balance between profitability and customer affordability.
By leveraging data analytics, companies like Airbnb have revolutionized the hospitality industry, optimizing income for hosts while maintaining competitive rates for guests.
Implementing dynamic pricing involves several best practices:
- Define Clear Objectives: Establish specific goals to guide pricing strategies.
- Ensure Data Quality: Base pricing adjustments on accurate and comprehensive data.
- Monitor and Adapt: Continuously review and refine pricing decisions to stay competitive.
- Share Insights: Facilitate informed decision-making by making data accessible across the organization.
It's crucial to prioritize data security to maintain integrity and compliance throughout the process. The table below summarizes the key steps:
Step | Description |
---|---|
1 | Define objectives for pricing strategy |
2 | Ensure high-quality data for analysis |
3 | Monitor market and adapt prices accordingly |
4 | Share insights for organizational alignment |
Best Practices for Implementing Data Analytics in Business Processes
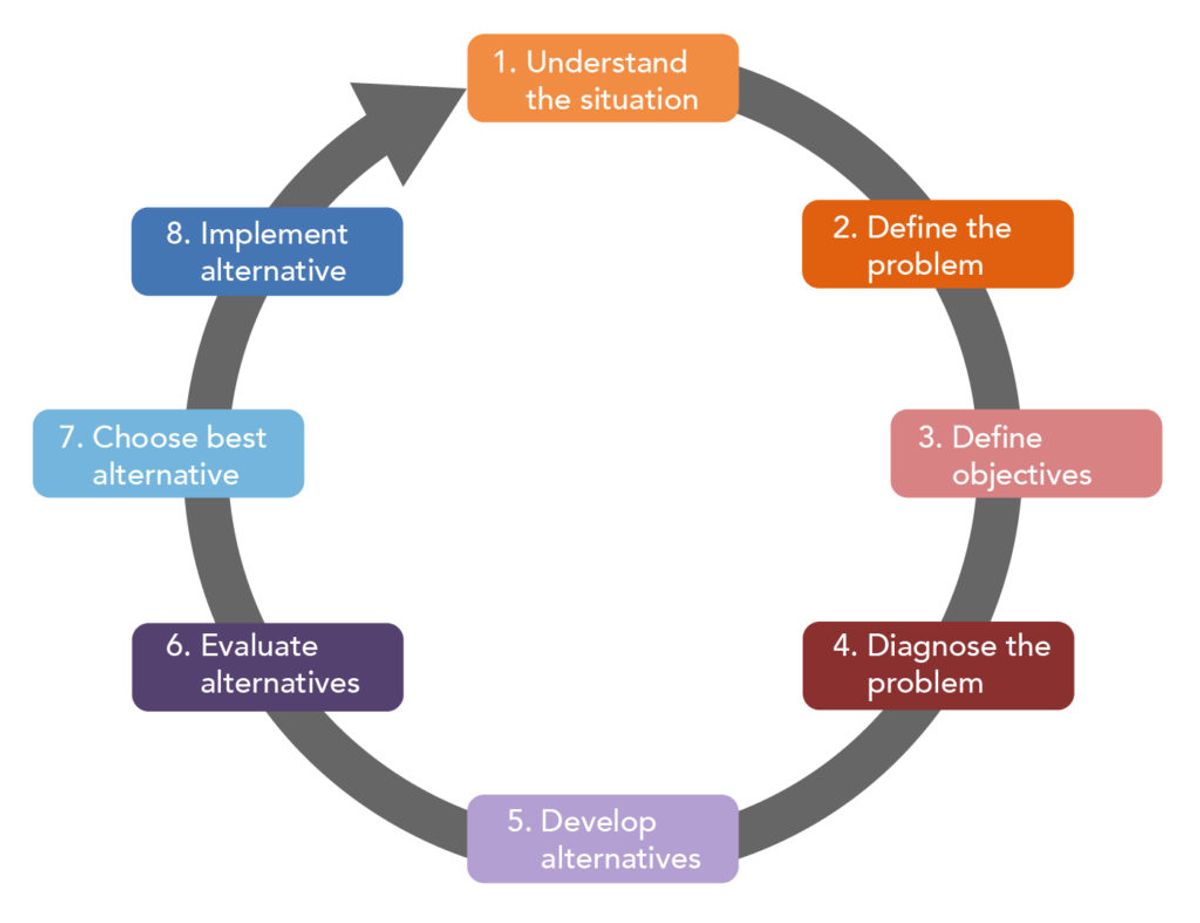
Establishing a Data-Driven Culture
To truly leverage analytics, organizations must foster a data-driven culture where decisions are anchored in empirical data rather than intuition. This involves setting clear goals, gathering relevant data, and investing in the right technology for data analysis. It's essential to develop the team's analytical skills and provide the necessary tools and training.
- Encourage employees to base decisions on data
- Set clear goals and gather relevant data
- Invest in technology for effective data analysis
- Develop analytical skills within the team
Iterate and experiment to drive a culture of continuous improvement. Use data to test ideas, measure impact, and make informed decisions based on the results. Communicate the benefits of data-driven decision making (DDDM), engage change advocates, and introduce pilot projects to illustrate its impact.
Organizations deeply rooted in data-driven practices are significantly more likely to experience substantial improvements in decision-making. The DDDM approach empowers businesses to anticipate challenges and act decisively.
Ensuring Data Quality and Integrity
The reliability of data-driven decisions hinges on the quality and integrity of the data utilized. Inaccurate or incomplete data can lead to erroneous conclusions and suboptimal business outcomes. To safeguard against these pitfalls, it's essential to implement robust measures for ensuring data accuracy and reliability.
One fundamental approach is to standardize data collection methods. This helps in maintaining consistency and minimizing errors. For instance, when collecting survey data, employing skip logic and validation rules can significantly reduce inaccuracies and inconsistencies.
- Perform Regular Data Quality Audits
- Verify Compliance with Data Governance Policies
- Ensure Data Quality Metrics are Met
Ensuring the quality, reliability, and timeliness of data is non-negotiable for credible analytics.
Verification of data sources is also crucial. It guarantees that the information used in the decision-making process is accurate and dependable. Regular audits of data and processes can identify areas for improvement and ensure adherence to data governance policies.
Integrating Analytics into Daily Operations
To remain competitive, businesses must weave analytics into the very fabric of their daily operations. Embedded analytics in global workflows allows users to access and analyze data within their regular work environments, fostering a seamless integration of insights into every business process. This approach not only enhances decision-making but also increases user engagement and streamlines data infrastructure.
Real-time analytics is a game-changer, providing immediate insights that can be acted upon swiftly. By embedding analytics tools into business operations, companies can quickly identify trends, customer preferences, and areas for improvement. Here are some key steps to effectively integrate analytics into daily operations:
- Establish unified data platforms for consistency across the organization.
- Implement real-time processing to capture and analyze data as it flows.
- Encourage the sharing of insights to promote collaborative decision-making.
By integrating analytics into strategic planning, businesses can boost their competitiveness and operational efficiency, leading to improved performance and profitability.
Success stories across various industries demonstrate the tangible benefits of this integration, with many reporting significant gains in efficiency and profitability.
Overcoming Common Obstacles in Data-Driven Decision Making
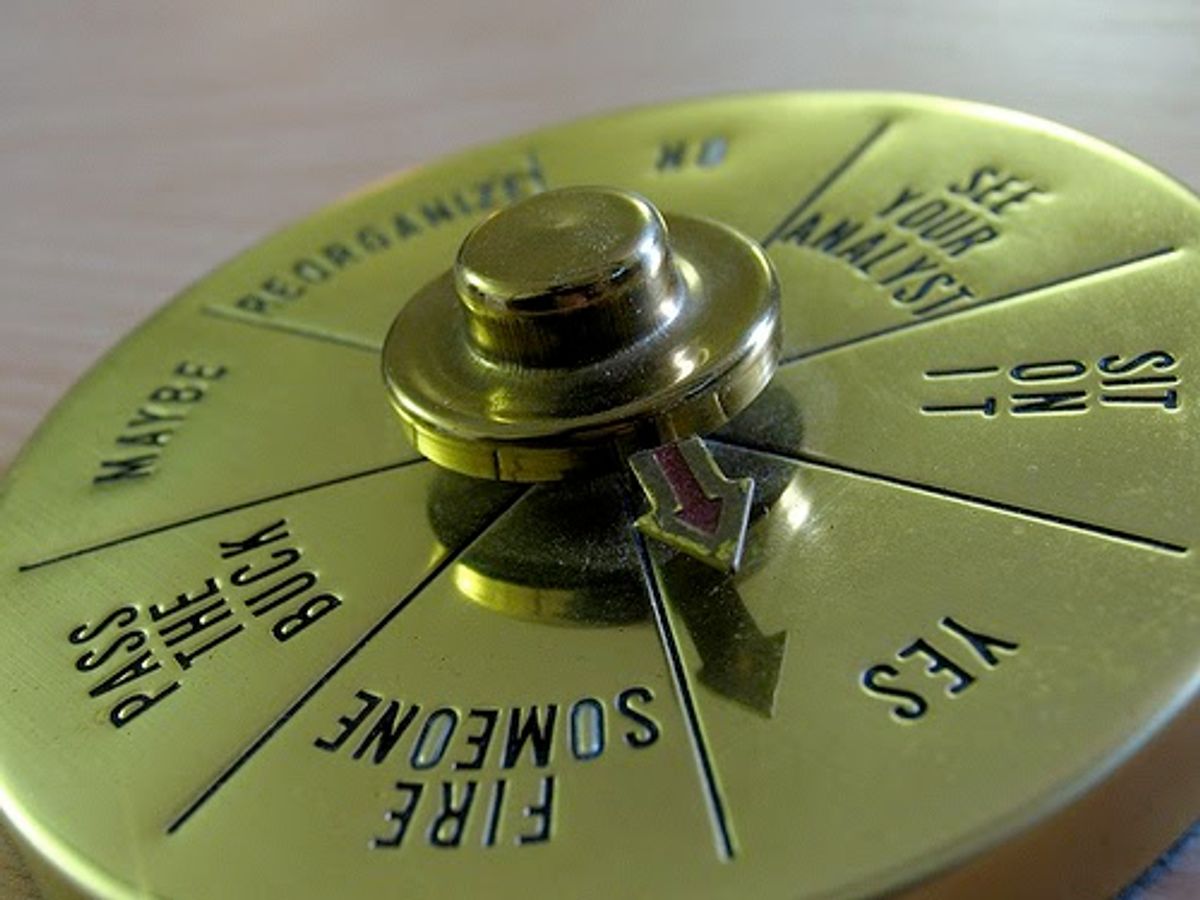
Addressing Data Privacy and Security Concerns
In the age of data-driven decision making, ensuring data privacy and security is not just a legal obligation but a cornerstone of customer trust. Cultivating a data-driven culture empowers employees with knowledge and tools for better decision-making. However, navigating challenges like privacy and ethics is crucial for safeguarding data and maintaining trust.
Ethical considerations in data usage are paramount. Businesses must balance the pursuit of insights with the responsibility of protecting individual privacy rights. This balance is essential to avoid reputational damage and the erosion of customer trust.
To achieve this, organizations should focus on:
- Establishing clear data governance policies to standardize, secure, and make data accessible.
- Implementing role-specific data literacy programs to enhance understanding of privacy implications.
- Engaging in human oversight to ensure responsible use of data, respecting the rights and dignity of individuals.
By addressing these concerns, businesses can navigate the complexities of big data while fostering an environment of trust and ethical practice.
Navigating the Complexity of Big Data
The realm of big data is a labyrinth of information, offering a treasure trove of insights that, when harnessed correctly, can propel businesses to new heights. Navigating this complexity is essential for companies aiming to stay competitive in a data-driven world. Big data best practices are the compass that guides organizations through this intricate environment, ensuring that data is used precisely, securely, and ethically.
Moreover, the ability to enhance products and services through data-driven insights is a game-changer for businesses. By analyzing customer data, companies move from guesswork to informed decision-making, which is critical for staying ahead of the curve. This process involves not just tracking customer reactions but also observing competitors and assessing market trends.
Advanced analytics tools and machine learning algorithms are at the forefront of tackling big data's complexity. They reveal patterns and relationships that might otherwise remain hidden, enabling more nuanced and strategic decisions.
As we look to the future, the importance of data analysis in strategic decision-making will only increase. However, businesses must also be aware of the potential pitfalls, such as misinterpretation of data, overreliance on numerical insights, and data privacy risks. Addressing these challenges head-on is crucial for reaping the long-term benefits of data-driven decision making (DDDM).
Avoiding Analysis Paralysis
Analysis paralysis is a critical obstacle in the realm of data-driven decision-making. When decision-makers face an overload of data, the risk of becoming immobilized by indecision or delayed actions is high. To combat this, it's essential to prioritize data based on its relevance to the company's strategic goals.
- Prioritize your choices
- Create your daily plan
- Automate your decision making
A balanced approach is crucial, blending data with industry knowledge to avoid the pitfalls of over-reliance on data. This means acknowledging that while data is a powerful tool, it is not the only factor in making informed decisions. Collaboration between data analysts and other professionals can lead to more holistic and effective outcomes, combining the precision of data with the wisdom of experience.
The goal of analysis should always be to derive actionable insights that align with predefined objectives. Interpreting complex data findings into understandable and relevant insights is vital for effective strategy formulation.
The Future of Decision Making: AI and Machine Learning in Analytics
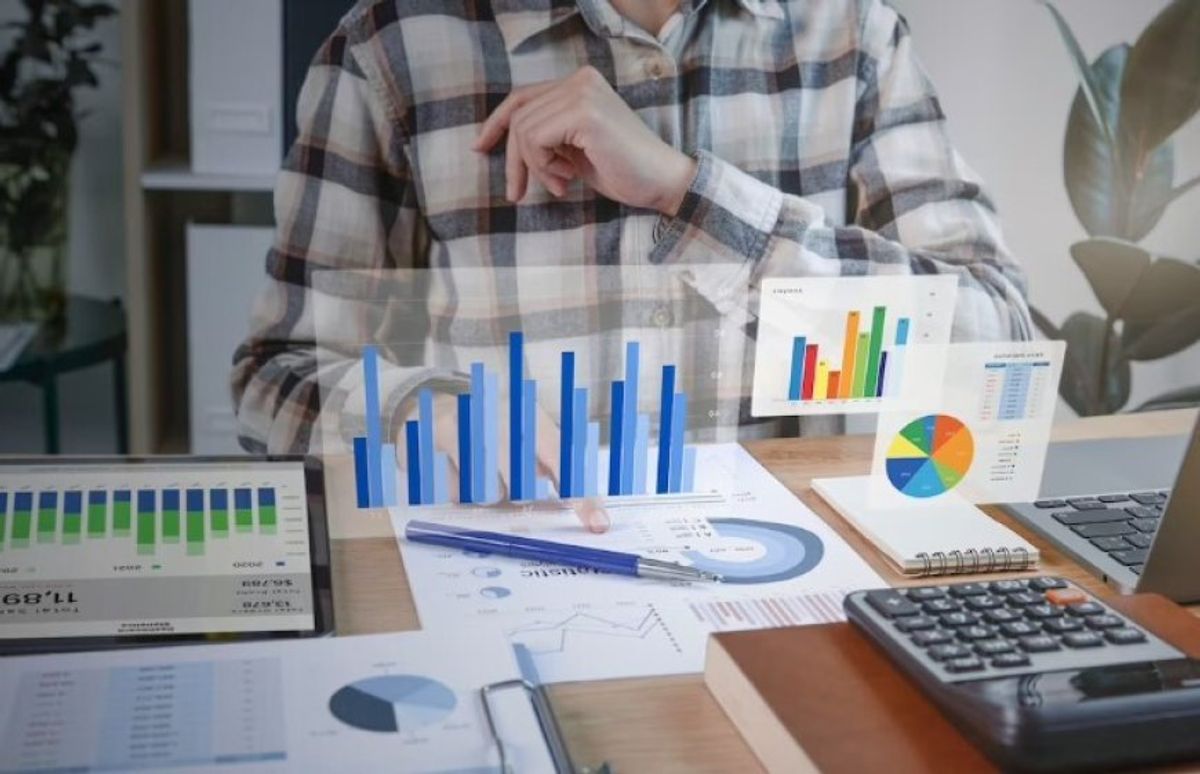
Automating Decision Processes with AI
The integration of artificial intelligence (AI) into decision-making processes marks a significant shift in how businesses approach analytics. AI revolutionizes analytics and decision-making in enterprise software, offering predictive insights, automated processes, and enhanced user experience through personalized interfaces and content.
AI's predictive capabilities enable organizations to anticipate challenges and seize opportunities, transforming raw data into strategic assets.
The benefits of AI in decision-making are manifold, including improved accuracy and efficiency, as well as the ability to process and analyze data at unprecedented speeds. Here are some key advantages:
- Improved Accuracy and Efficiency: AI algorithms can process data faster and more accurately than humans, reducing the chances of errors and biases.
- Predictive Capabilities: AI can analyze data to find patterns and predict future trends.
By adopting an approach of incremental decision-making, organizations can manage the complexity of data-driven decisions more effectively. This involves making preliminary decisions based on available data and refining those decisions as more information becomes available or as outcomes are evaluated.
Enhancing Predictive Models with Machine Learning
The integration of machine learning (ML) into predictive analytics marks a significant leap forward in a business's ability to forecast and adapt to future trends. Advanced ML algorithms are pivotal in revealing patterns and insights that traditional analysis might miss, offering a competitive edge in strategic planning.
- Predictive Analytics: Leverages historical data to anticipate market demands and customer behavior.
- Hidden Insights: ML uncovers relationships in data, enhancing decision-making.
- Continuous Improvement: Enables ongoing optimization of business processes.
By harnessing the power of machine learning, organizations can refine their predictive models, ensuring they remain relevant and accurate in a rapidly changing environment.
It's essential to address potential pitfalls such as lookahead bias, which can distort the model's accuracy. Segregating training and validation data is a critical step in preventing this issue and maintaining the integrity of predictive insights.
Ethical Considerations in Automated Decision Making
As organizations increasingly rely on automated systems for decision making, the ethical implications of AI become a critical concern. Ensuring the responsible use of AI is not just a technical challenge but a moral imperative. Ethical considerations must be woven into the fabric of AI systems to safeguard against unintended consequences.
Transparency in AI processes is essential for maintaining trust and accountability. It is important for stakeholders to understand how decisions are made and to ensure that AI systems do not perpetuate biases or infringe on privacy rights. The following points highlight key ethical considerations:
- The necessity for human oversight to respect the rights and dignity of individuals.
- Balancing the benefits of data utilization with the protection of individual privacy.
- Establishing clear guidelines for data security to prevent misuse.
The integration of ethical principles in AI decision making is not just a compliance issue but a cornerstone of sustainable and trustworthy business practices.
The table below summarizes the ethical challenges and proposed measures in AI decision making:
Ethical Challenge | Proposed Measure |
---|---|
Bias and Fairness | Implement regular audits |
Privacy | Enforce strict data governance |
Accountability | Develop clear AI ethics policies |
Addressing these ethical considerations is imperative for businesses that wish to remain competitive and retain customer trust in an era where AI plays a pivotal role in strategic decisions.
The Role of Visualization in Communicating Data Insights
Choosing the Right Visualization Tools
Selecting the appropriate visualization tools is crucial for effectively communicating complex data insights. The right tools can transform raw data into clear, actionable information, enabling decision-makers to grasp intricate patterns and relationships at a glance. When evaluating tools, consider the following aspects:
- Ease of use: Tools should have intuitive interfaces that allow users to create and interpret visualizations without extensive training.
- Functionality: Look for features like interactive dashboards, real-time data updates, and the ability to handle large datasets.
- Compatibility: Ensure the tools integrate seamlessly with your existing data systems and workflows.
Tableau and Power BI are examples of platforms that offer advanced data visualization capabilities, allowing users to create interactive dashboards and reports from multiple data sources. These tools are particularly valuable for businesses requiring complex data analysis and visualization.
It's essential to assess your business needs and goals before choosing a tool. Consider the data type and volume, and your team's technical skills.
Finally, don't overlook the importance of scalability, integration, and support. As your business grows, your data visualization needs will evolve. The tools you select should be able to grow with you, providing ongoing support and updates. Research and reviews can offer insights into tool performance, but consulting with experts can lead to tailored recommendations that empower your business to extract insights and make smarter, data-driven decisions.
Designing Dashboards for Decision Support
Effective dashboards are a cornerstone of data-driven decision-making, providing a visual interface that encapsulates critical information at a glance. Building a data-literate culture requires ongoing commitment, and dashboards play a pivotal role in this endeavor. They must be intuitive and align with the audience's knowledge level, ensuring that insights are accessible and actionable.
To achieve this, consider the following best practices:
- Align visualizations with the audience's knowledge level
- Use design elements judiciously to avoid clutter
- Ensure that the dashboard is interactive, allowing users to drill down into the data
Successes and pitfalls in data-driven decision-making highlight the importance of leveraging insights wisely.
Remember, the goal is not just to present data, but to tell a story that leads to informed decisions. Evaluation of dashboards should be an ongoing process, providing critical feedback that informs future enhancements and keeps the tool relevant and effective.
Storytelling with Data
In the realm of business intelligence, storytelling with data stands out as a transformative force. It's not just about presenting numbers; it's about weaving a narrative that resonates with stakeholders and drives action. By leveraging data-driven strategies, decision-makers can illuminate insights that would otherwise remain obscured in spreadsheets and reports.
Effective data storytelling often involves the use of visualization techniques. For instance:
- A line chart illustrating the evolution of customer satisfaction over time
- A bar chart showcasing comparative performance across product categories
These visual aids are not mere embellishments; they are essential tools that enhance comprehension and facilitate strategic decision-making.
The art of data storytelling is about simplifying the complex without losing the nuanced details that inform smart business moves.
As we empower decision-making with BI tools, it's crucial to remember that data stories are not just for data scientists. They should be accessible to all levels of an organization, fostering a culture where data literacy is the norm and insights lead to growth.
Building a Scalable Analytics Framework for Growth
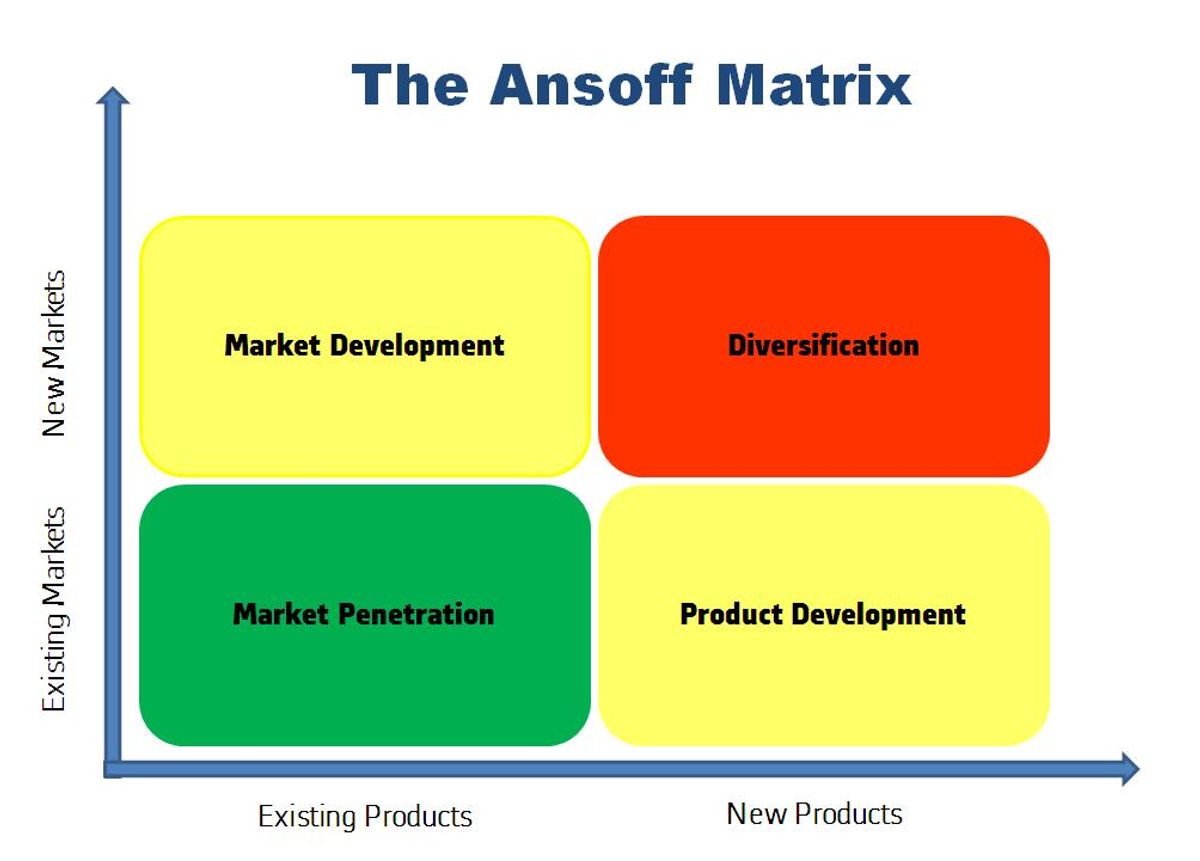
Adapting Analytics Strategies for Business Expansion
As businesses grow and enter new markets, the role of data analytics becomes increasingly critical. Scalability and flexibility are essential attributes of successful analytics strategies, particularly when expanding internationally. Adapting these strategies allows for the identification of customer acquisition channels and the understanding of diverse cultural preferences, which are pivotal for global market penetration.
- Identifying and repairing conversion funnel leaks to enhance website conversion rates
- Tailoring campaigns to resonate with global audiences
- Innovating products to meet international market demands
- Assessing risks in international operations
The adaptability provided by data analytics is crucial in the global market, helping businesses innovate and adjust their offerings based on changing consumer preferences and market dynamics.
By leveraging data analytics, companies can develop innovative products and services that cater to the nuanced needs of different regions. This not only aids in staying ahead of competitors but also ensures a more personalized approach to customer service, which is key to customer retention in international markets.
Leveraging Cloud Computing for Analytics Scalability
The adoption of cloud computing has revolutionized the scalability of data analytics, offering businesses the flexibility to expand their data processing capabilities as needed. Cloud services provide a cost-effective and efficient way to handle large volumes of data, ensuring that analytics can keep pace with business growth without the need for significant upfront investment in infrastructure.
Scalability is a critical advantage of cloud computing, allowing organizations to adjust their resources in response to fluctuating data demands. This elasticity means that companies can scale up during peak times and scale down when demand wanes, optimizing costs and maintaining performance.
By leveraging cloud computing, businesses can focus on extracting insights and driving value from their data, rather than worrying about the underlying infrastructure.
Here are some key considerations when leveraging cloud computing for analytics scalability:
- Assessing current and future data storage and processing needs.
- Choosing a cloud provider with a robust suite of analytics tools and services.
- Ensuring seamless integration with existing data sources and systems.
- Evaluating the security measures and compliance standards of the cloud service.
- Planning for a smooth transition to the cloud, minimizing disruption to operations.
Fostering Innovation through Data Exploration
In the realm of business growth, data exploration is a pivotal activity that fuels innovation. By delving into data, companies can uncover hidden patterns, market trends, and customer preferences that inform product development and strategic initiatives. Innovation thrives when organizations leverage data to drive a culture of experimentation and continuous improvement.
Data exploration is not just about gathering information; it's about asking the right questions and being willing to follow where the data leads.
To effectively harness data for innovation, businesses should:
- Collect relevant data that aligns with product goals and objectives.
- Analyze and visualize data to extract meaningful insights.
- Iterate and experiment with new ideas, measuring their impact and refining based on data-driven feedback.
These practices ensure that data exploration is not a one-time event but an ongoing process that contributes to a sustainable competitive advantage.
Cultivating Data Literacy Among Decision Makers
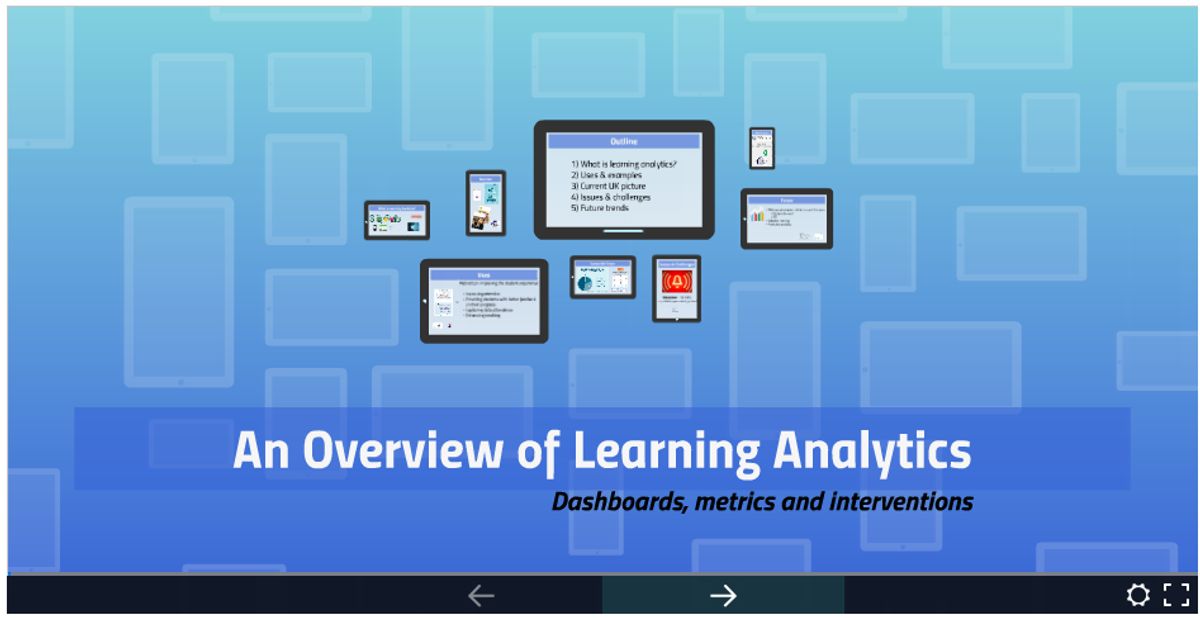
Training Programs for Non-Technical Executives
In the journey towards a data-centric business culture, training programs for non-technical executives are pivotal. These programs are designed to bridge the skills gap in data science and analytics, empowering leaders to make informed decisions based on data insights.
- Training Needs Analysis: Identify the specific data competencies required for executive roles.
- Learning Objectives: Define what executives should be able to do with data post-training.
- Curriculum Design: Develop a tailored curriculum that addresses the unique needs of non-technical leaders.
- Training Implementation: Deliver the training using methods that resonate with executive learning styles.
- Program Evaluation: Assess the effectiveness of the training and its impact on decision-making.
By fostering a collaborative data-driven environment, these programs help overcome challenges in data-driven decision-making and cultivate leadership for a data-first approach. It's not just about learning to use tools like Tableau or Microsoft AI, but about transitioning to a mindset where data is integral to strategy and operations.
Promoting a Culture of Continuous Learning
In the realm of data analytics, promoting a culture of continuous learning is pivotal for maintaining a competitive edge. Fostering a culture of continuous learning and improvement is essential, as it encourages experimentation, reflection, and adjustment. This iterative process is not just about collecting data, but also about applying insights to drive better business outcomes.
To embed this culture, organizations should:
- Encourage experimentation with new ideas and strategies.
- Measure the impact of these experiments and use data to inform decisions.
- Reflect on both successes and failures to refine future strategies.
Embracing a feedback loop is crucial for ensuring that data-driven decision making becomes an ongoing practice, setting the stage for continuous improvement and adaptation.
By continuously assessing and refining strategies based on new data and feedback, businesses can adapt more swiftly to market changes and customer needs. This approach not only aligns decisions with business objectives but also fosters an environment where learning is an integral part of the operational ethos.
Bridging the Gap Between Data Teams and Business Units
Breaking down data silos is just the first step. Encouraging collaboration between data analysts and other departments ensures a richer, more nuanced understanding of data. When teams from different functional areas come together, they can provide diverse perspectives that enhance the interpretation and application of data.
A balanced approach to data-driven decision-making involves integrating data analysis with human insight, industry expertise, and an understanding of the broader market context.
Ask Yourself: How can we ensure that the insights gained are effectively translated into actionable strategies, and who will be responsible for overseeing this process?
The transition to DDDM (Data-Driven Decision Making) is not without its challenges. Here's a streamlined approach to address them:
- Data Silos
- Solution: Centralize data with integrated platforms to enhance sharing and collaboration across departments.
- Lack of Data Literacy
- Solution: Implement training programs to improve data literacy across all levels of the organization.
They foster environments where data analysts work closely with other professionals, sharing insights and perspectives. This collaboration leads to more holistic, informed, and effective decision-making, blending the precision of data with the wisdom of experience.
Evaluating the Return on Investment for Analytics Initiatives
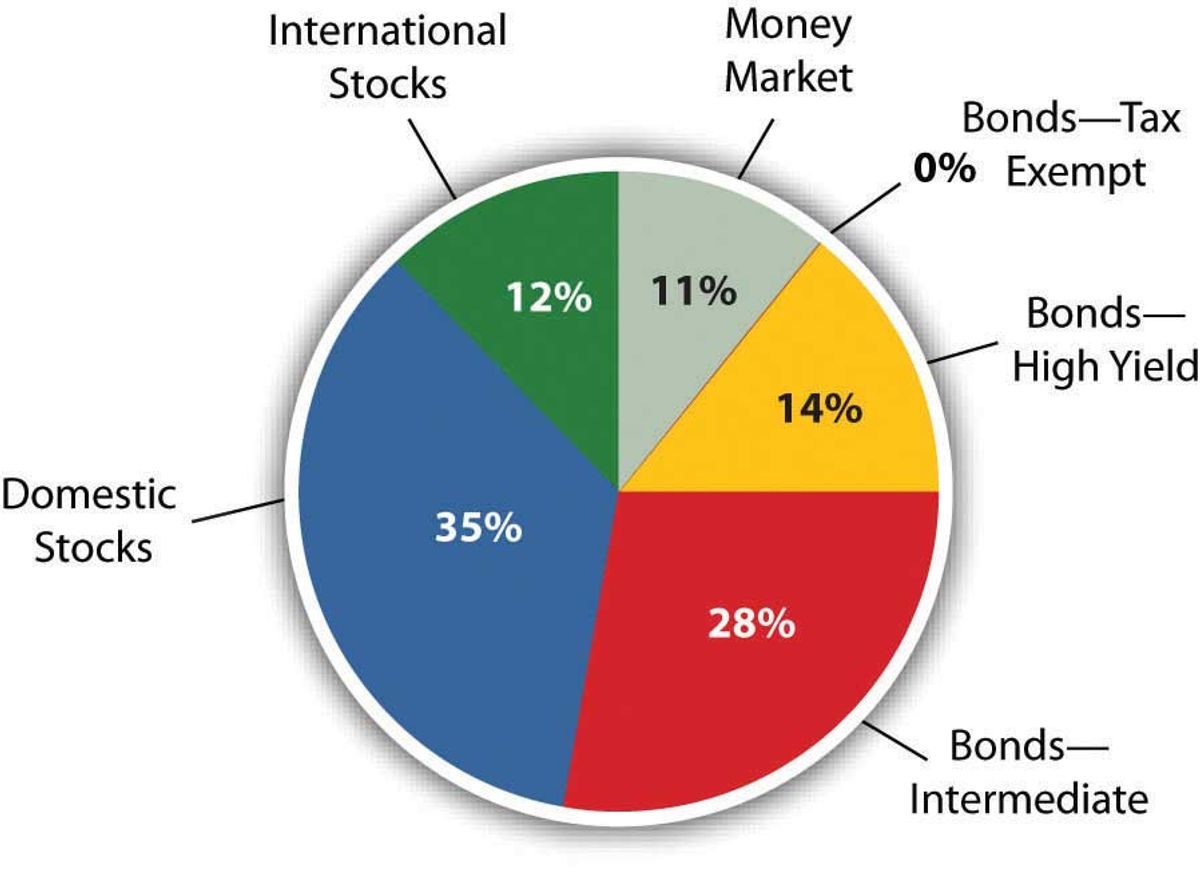
Assessing the Financial Impact of Analytics
To truly understand the financial benefits of data analytics, businesses must look beyond the surface and quantify the impact on their bottom line. Real-time analytics drive improvements in key performance indicators (KPIs), which are essential for measuring success in various aspects of business operations. For instance, analytics can lead to enhanced operational efficiency by identifying areas where costs can be reduced or productivity can be increased.
The strategic use of analytics enables businesses to set SMART financial goals, ensuring precision in target setting and effectiveness in progress monitoring.
Here's a snapshot of common KPIs affected by data analytics:
KPI | Description |
---|---|
ROI | Financial returns from analytics initiatives |
Customer Satisfaction | Effectiveness of data-driven decisions |
Operational Efficiency | Cost reductions and productivity gains |
Market Share | Impact on competitive positioning |
Each of these KPIs provides a lens through which the financial impact of analytics can be viewed, offering a clearer picture of the return on investment for analytics initiatives.
Calculating Cost Savings and Revenue Growth
To effectively evaluate the ROI of analytics initiatives, organizations must translate data insights into quantifiable financial metrics. Calculating cost savings and revenue growth is pivotal in demonstrating the tangible benefits of data-driven strategies. By focusing on key areas such as operational efficiency and customer satisfaction, businesses can measure the direct impact of analytics on their bottom line.
Operational efficiency, for example, can be tracked through metrics like reduced operational costs or increased productivity. Similarly, customer satisfaction can be monitored to gauge the effectiveness of data-driven decisions on client retention and acquisition. The table below succinctly captures these key metrics:
Metric | Description |
---|---|
ROI | Financial impact of data analytics initiatives |
Customer Satisfaction | Effectiveness of data-driven decisions |
Operational Efficiency | Improvements in costs and productivity |
Market Share | Impact on competitive positioning |
Emphasizing the importance of these metrics ensures that data analytics is not just a theoretical exercise but a practical tool for enhancing financial performance.
The correlation between meticulous data analysis and financial outcomes is undeniable. Research indicates that even a 1% increase in conversion rates can significantly boost annual revenue. Moreover, improving customer retention rates by 5% can lead to profit increases between 25% to 95%, showcasing the profound effect of data analytics on an organization's growth trajectory.
Long-Term Strategic Benefits of Data-Driven Decisions
The adoption of data-driven decision-making is a transformative strategy that can redefine a company's trajectory. Big data integration is often the cornerstone of success stories in enhancing operational efficiency and strategic foresight. Over time, organizations that leverage data effectively can expect a multitude of benefits that transcend immediate financial gains.
- Strategic agility: Data empowers businesses to pivot quickly in response to market changes.
- Customer centricity: Insights from data analytics drive a deeper understanding of customer needs.
- Innovation: A data-rich environment fosters creativity and new solutions.
The strategic insights gained from analytics are not just about the numbers; they're about shaping a future-proof business.
Challenges such as ensuring data quality and building robust analytics capabilities are part of the journey. However, the long-term strategic benefits of integrating analytics into business strategy are clear. They lay the groundwork for a culture of informed decision-making that supports sustainable growth and competitive advantage.
Maximizing the value of your data is crucial in today's competitive landscape, and understanding the return on investment (ROI) for analytics initiatives is key to strategic decision-making. At OptimizDBA, we specialize in database optimization consulting, ensuring that your data solutions are not just faster, but also more efficient and reliable. Our proprietary methodologies have consistently delivered performance increases, often by orders of magnitude. Don't just take our word for it; our track record of enhancing transaction speeds and our long list of satisfied clients since 2001 speaks volumes. Ready to unlock the full potential of your data? Visit our website to learn how we can transform your analytics initiatives into a competitive advantage.
Conclusion
In the journey towards data-driven excellence, businesses must embrace the transformative power of analytics to inform their decision-making processes. The insights gleaned from this article underscore the indispensable role of data analytics in enhancing business decisions, identifying growth opportunities, and maintaining a competitive edge. By adhering to best practices, such as leveraging advanced analytics techniques and embedding analytics into daily operations, organizations can optimize their strategies and achieve superior outcomes. As we've seen with examples like Netflix, the application of data analytics is not just a trend but a cornerstone of modern business strategy. In conclusion, companies that effectively harness the potential of data analytics are poised to lead their industries, innovate relentlessly, and deliver unparalleled value to their customers.
Frequently Asked Questions
How does data analytics enhance business decision-making?
Data analytics enhances business decision-making by providing insights from various data sources, enabling companies to identify market trends, optimize pricing, improve customer satisfaction, and make fact-based choices rather than relying on assumptions.
What are the risks and challenges of data-driven decision-making?
The risks include potential flaws in data analysis leading to inaccurate conclusions. Challenges encompass handling massive data volumes, ensuring data quality, and avoiding analysis paralysis where decision-making is stalled due to overanalyzing data.
How can businesses measure the impact of data analytics on performance?
Businesses can measure the impact of data analytics by tracking Key Performance Indicators (KPIs), interpreting analytics results, and benchmarking against industry standards to assess improvements in efficiency, productivity, and customer experience.
What are advanced analytics techniques for predictive insights?
Advanced analytics techniques include predictive analytics, which forecasts future outcomes like customer demand, and prescriptive analytics, which suggests optimal decisions such as pricing strategies to maximize profitability.
What are the best practices for implementing data analytics in business?
Best practices include establishing a data-driven culture, ensuring data quality and integrity, and integrating analytics into daily operations to make informed strategic decisions and gain a competitive edge.
What common obstacles do businesses face in data-driven decision-making?
Common obstacles include addressing data privacy and security concerns, navigating the complexity of big data, and avoiding analysis paralysis by ensuring data analysis leads to actionable insights rather than endless deliberation.
How will AI and machine learning change future decision-making?
AI and machine learning will automate decision processes, enhance predictive models, and introduce ethical considerations as automated systems increasingly influence business strategies and outcomes.
What role does data visualization play in decision-making?
Data visualization plays a crucial role in communicating insights by helping decision-makers understand complex data through intuitive graphical representations, supporting the use of dashboards for decision support, and storytelling with data.