The Role of Predictive Analytics in Modern Business Strategies
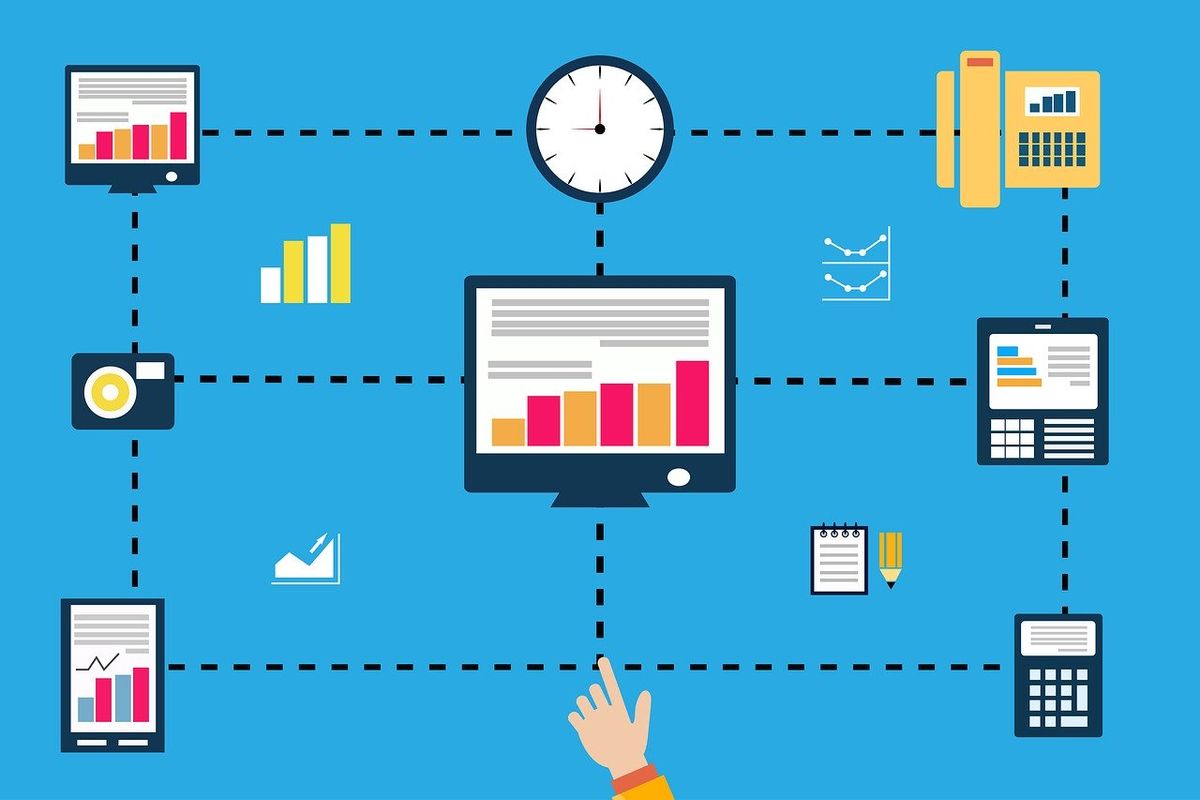
Understanding Predictive Modeling
At the heart of predictive analytics lies predictive modeling, a process that turns historical data into valuable insights for future decision-making. Predictive modeling focuses on creating models to make predictions, enhancing decision-making accuracy and optimizing resource allocation. It's a cornerstone that transforms raw data into a strategic asset.
Adopting predictive modeling in business is not just about having the right tools; it's about integrating these insights into actionable strategies. As models mature, they become indispensable, providing foresight that drives engagement and competitive advantage.
Building a predictive model is a multi-layered process, starting with data collection and culminating in the seamless integration of these models into business workflows. This integration ensures that businesses are proactive rather than reactive, maintaining a strategic edge.
Here's a brief overview of the predictive modeling process:
- Data Collection: Gathering relevant historical data.
- Model Building: Using statistical techniques to create predictive models.
- Validation: Testing the model to ensure accuracy.
- Implementation: Integrating the model into decision-making processes.
- Continuous Learning: Updating the model with new data to improve predictions.
Enhancing Competitive Advantage
In the quest to remain ahead of the curve in a competitive market, businesses are increasingly turning to predictive analytics. This powerful tool allows companies to not only understand current trends but also to anticipate future market movements. By analyzing competitor performance and monitoring industry trends, organizations gain valuable market intelligence that can be used to outmaneuver rivals.
Predictive analytics serves as a catalyst for enhanced profitability. It enables businesses to optimize operational efficiencies, refine supply chains, and develop marketing strategies that resonate with their target audience, ultimately boosting revenue streams.
Furthermore, predictive analytics aids in identifying high-value customers, allowing companies to focus their efforts on nurturing loyalty and maximizing long-term value. The table below illustrates the key areas where predictive analytics can enhance competitive advantage:
Area of Impact | Description |
---|---|
Operational Efficiency | Fine-tuning processes to reduce costs and increase productivity. |
Supply Chain Optimization | Anticipating demand to streamline inventory and delivery. |
Marketing Strategy | Tailoring campaigns to effectively engage target demographics. |
By leveraging precise analytics, businesses are not just reacting to the market; they are proactively shaping their future, ensuring they stay ahead in a highly competitive and ever-evolving market landscape.
Case Studies: Strategic Success Stories
The application of predictive analytics in business has led to numerous success stories across various sectors. Data analytics case studies with solutions provide insights that empower leaders to make strategic choices backed by evidence, leading to improved decision-making and growth. For instance, in marketing, informed decisions have led to greater impact through targeted campaigns and optimized customer journeys.
Embrace the data, embrace the journey, and watch your business thrive.
In the realm of supply chain management, predictive analytics has been instrumental in forecasting demand, managing inventory, and reducing costs. The following list highlights key areas where predictive analytics has been successfully applied:
- Corporate Governance
- Innovation
- Finance
- Marketing
- Supply Chain
- Organizational Learning
- Luxury
Each case study serves as a testament to the transformative power of data-driven strategies in the modern business landscape.
Data-Driven Decision Making: From Insight to Action
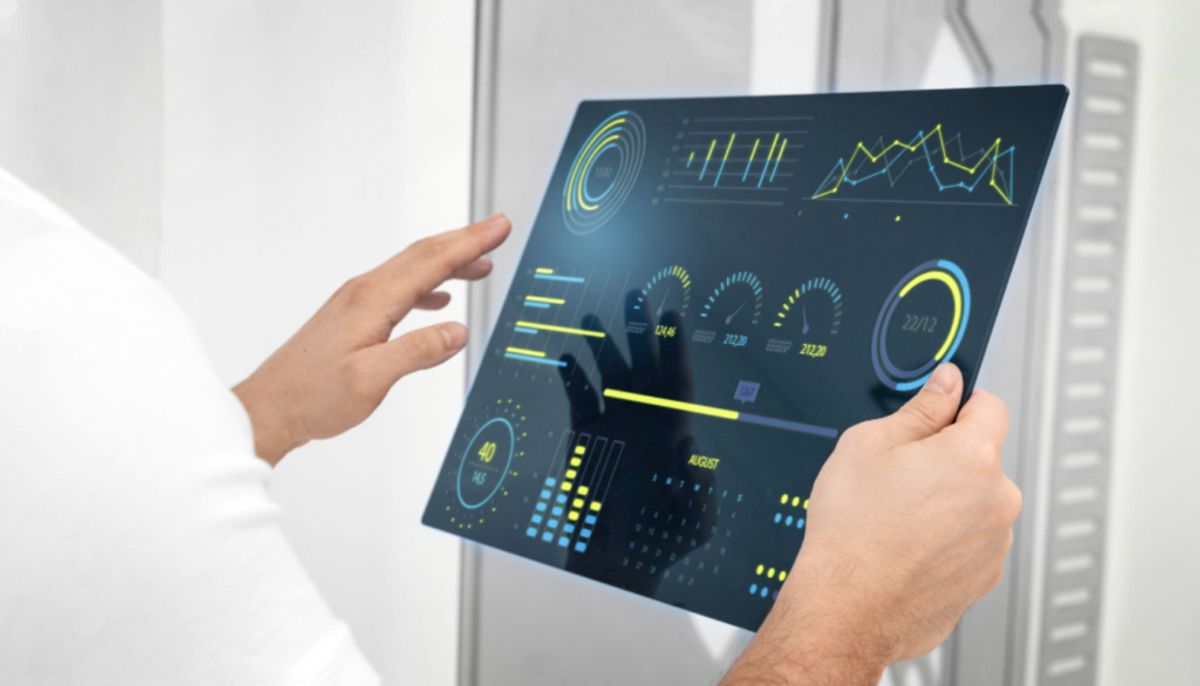
Translating Data into Strategic Moves
Collecting data is the first step in a journey that culminates in the mastery of strategic decision-making. The true value of data lies in its translation into actionable insights that can pivot a business towards success. Machine learning algorithms are particularly adept at sifting through data to reveal patterns and predictions, empowering businesses to adjust their strategies in real time.
In the realm of business, data serves as a strategic compass, guiding companies to informed, profitable, and risk-averse decisions.
The process of transforming data into strategic moves involves several key steps:
- Identifying the relevant data points
- Analyzing the data to uncover trends
- Formulating strategic actions based on insights
- Implementing the actions and monitoring outcomes
These steps ensure that data does not remain static but becomes a dynamic force driving business growth and innovation. Case studies across various industries have demonstrated the transformative impact of predictive analytics on profitability, showcasing the power of data-driven decision-making.
Real-Time Adaptation and Responsiveness
In the realm of business, the capacity for real-time adaptation and responsiveness is a game-changer. Predictive analytics tools empower proactive decision-making, allowing companies to adjust their strategies swiftly in response to emerging data. This agility is crucial for maintaining relevance and impact in a market where consumer behaviors and preferences shift rapidly.
With predictive analytics, businesses can ensure continuous monitoring and adjustment of their strategies, leading to enhanced operational efficiency and strategic planning.
Real-time bidding (RTB) in advertising technology is a prime example of predictive analytics at work. By leveraging algorithms that analyze user behavior and ad performance metrics, companies can optimize their ad placements for maximum engagement. This not only improves the return on investment but also ensures that marketing messages resonate effectively with the target audience.
- Analyzing and adjusting strategy in real-time
- Measuring ROI through marketing data analysis
- Guaranteeing continuous monitoring and adjustment
- Predictive campaign optimization
The Importance of Data Quality
The quality of data is the cornerstone of any predictive analytics endeavor. High-quality data is essential for generating accurate and actionable insights. It is a measure of a data set's condition based on factors such as accuracy, completeness, consistency, reliability, and validity. Without these characteristics, data can mislead and result in poor decision-making.
Investing in robust data management practices is crucial. Techniques such as standardization, validation, and de-duplication ensure that the data used in business intelligence (BI) is reliable and valuable. Regular data audits and data cleansing techniques are also vital components of maintaining data integrity.
The strategic prowess embedded in quality data analysis is a linchpin in elevating business strategies, amplifying profitability, and curtailing risks.
Ultimately, the effectiveness of BI and advanced analytics is contingent upon the quality of the underlying data. Real-time data can provide instant feedback on a marketing campaign's success, but only if it is accurate, clean, and structured. Thus, the focus on data quality is not just about number-crunching; it's about leveraging data as a strategic compass, guiding towards informed, lucrative, and risk-averse business decisions.
The Impact of Machine Learning on Business Forecasting
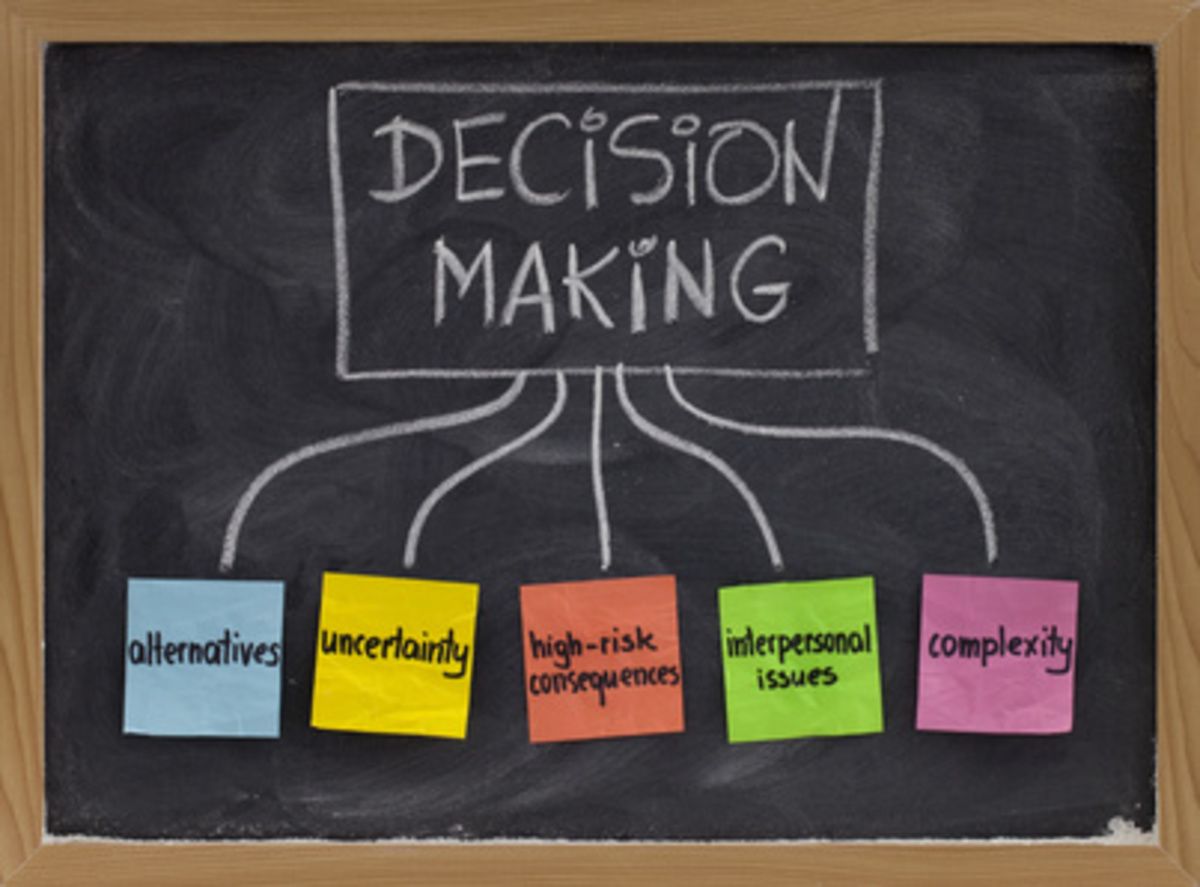
Uncovering Hidden Patterns
The advent of predictive analytics has revolutionized the way businesses approach their data, transforming it into a strategic asset. Analysts use statistical algorithms and machine learning to uncover the trends hidden within complex datasets and use them to predict future customer behavior, which is crucial for forecasting and risk management. This process of decoding complex data with advanced visualization tools is not just about gathering information; it's about peering through a magnifying glass at the intricate patterns that drive customer actions.
Unlocking the power of data and analytics provides unparalleled insight into customer motivations. By identifying key variables and insights, businesses can embark on a journey of exploration through their data, selecting the right modeling techniques to drive the success of targeted campaigns. Visualization tools are particularly effective in transforming numbers into meaningful stories, allowing companies to make informed decisions that propel them forward.
To truly unlock the power of data, one must not only collect but also interpret and analyze it. Look for patterns, trends, and correlations. It's the deep dive into data that uncovers hidden gems and informs strategic decision-making.
Improving Operational Efficiency
Operational efficiency is the cornerstone of a thriving business. By integrating machine learning with predictive analytics, companies are able to enhance forecasting, automate routine tasks, and make proactive decisions. This synergy is pivotal in refining business intelligence processes, leading to a more agile and responsive operational framework.
Business Intelligence (BI) empowers organizations to identify and rectify inefficiencies and bottlenecks. Detailed data analysis enables the streamlining of workflows, effective resource allocation, and the identification of improvement areas. The result is a significant boost in efficiency and cost savings, which ultimately enhances customer service.
The automation of operational processes liberates time for decision-makers to concentrate on strategic initiatives. With access to quicker and more accurate insights, executives can make well-informed decisions, optimizing business outcomes and driving profitability.
By leveraging precise analytics, businesses can fine-tune their operational efficiencies, optimize supply chains, and tailor marketing strategies to better connect with their target demographics, thus boosting revenue streams.
Predictive Analytics in Supply Chain Management
In the realm of supply chain management, predictive analytics is a game-changer, enabling companies to foresee and navigate the complexities of global logistics. Forecasting demand and inventory levels becomes more accurate, reducing the risk of overstocking or stockouts. By analyzing patterns in historical data, businesses can optimize their inventory, leading to significant cost savings and improved customer satisfaction.
- Anticipate supplier delays and adjust procurement schedules
- Predict maintenance needs to minimize equipment downtime
- Enhance route planning for shipping to reduce costs and delivery times
Predictive analytics empowers businesses to act rather than react, transforming supply chain operations from reactive to proactive management.
The integration of machine learning models further refines these predictions, allowing for real-time adjustments in response to market changes or disruptions. This strategic application of analytics not only streamlines operations but also provides a competitive edge in today's fast-paced market.
Optimizing Marketing Strategies with Predictive Analytics
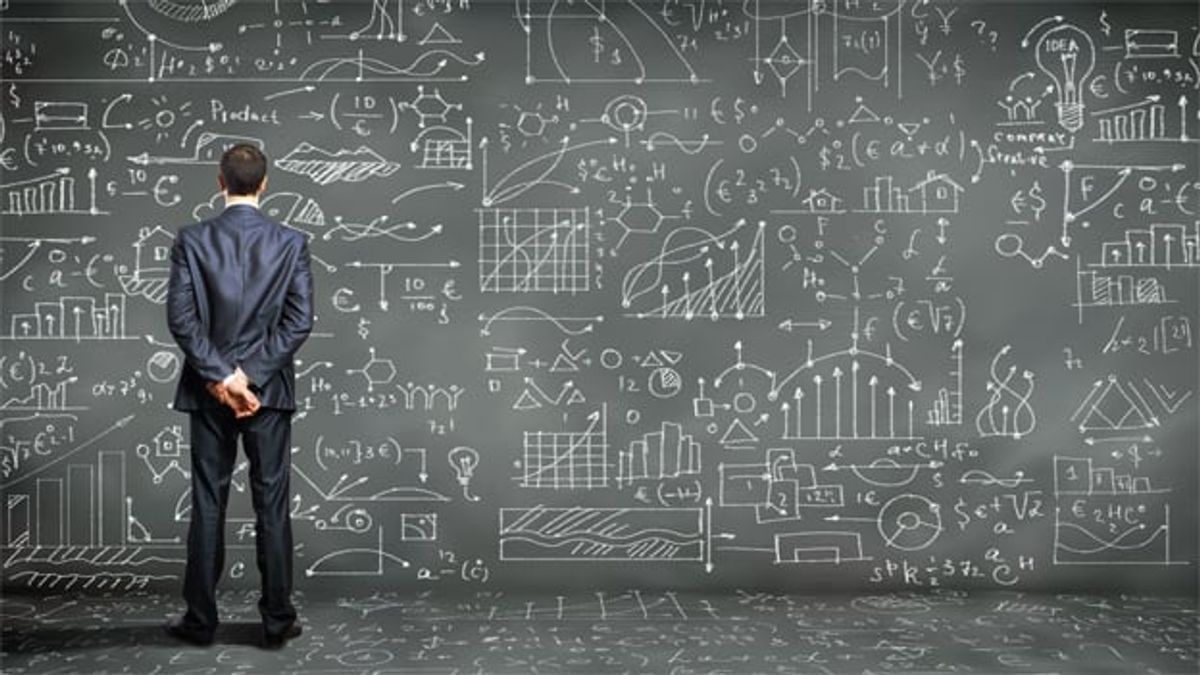
Enhancing Customer Journey Analysis
Gone are the days when we relied on guesswork and assumptions to understand the customer journey. Today, we have powerful tools and techniques that allow us to unlock the potential of data and revolutionize our understanding of how customers interact with our brand. From the very first moment of awareness to the ultimate satisfaction after purchase, data analytics empowers us to make informed decisions, optimize our strategies, and cultivate lasting relationships with our customers.
Optimizing customer experiences also involves enhancing customer satisfaction. Data analysis can unveil the touchpoints where customers might encounter frustrations or roadblocks. By identifying these pain points, you can proactively address them, streamline processes, and elevate the overall customer journey.
So, as you navigate the customer journey, keep a keen eye on these key touchpoints. Embrace data analysis as your compass, guiding you toward a deeper understanding of your customers' behavior, preferences, and needs. The role of predictive analytics in customer acquisition is pivotal, offering targeted marketing, increased efficiency, and improved customer engagement.
Maximizing Return on Investment
Integrating advanced analytics in marketing strategies not only enhances decision-making and adaptability but also significantly boosts ROI. Predictive insights enable tailored campaigns, which lead to more effective customer engagement and proactive adjustments, providing a competitive edge.
Enhanced customer experience is a direct result of personalized and relevant interactions across all touchpoints. By focusing on metrics that matter, businesses can justify marketing expenditures and demonstrate their contribution to the company's bottom line. This strategic approach to marketing ensures that resources are allocated towards high-value customers, nurturing their loyalty and maximizing their long-term value.
By leveraging precise analytics, businesses can fine-tune their operational efficiencies, optimize supply chains, and tailor marketing strategies that resonate well with their target demographics, thus boosting revenue streams.
Identifying the proper key performance indicators (KPIs) for your predictive analytics campaigns is crucial. These KPIs should align with your overarching business objectives to ensure that every marketing effort is a step towards enhanced profitability.
Real-World Applications in Marketing
In the realm of marketing, predictive analytics has become a game-changer, enabling businesses to optimize decision-making and tailor their campaigns to meet the evolving demands of the market. By leveraging machine learning, companies can engage in hyper-personalization, crafting messages and offers that resonate deeply with individual customer needs. This approach not only enhances customer satisfaction but also sharpens competitive strategies.
- Hyper-personalization of marketing messages
- Dynamic pricing strategies
- Product recommendation engines
The agility afforded by predictive analytics allows for real-time adaptation of marketing strategies. As consumer behaviors shift, so too can the messaging, ensuring relevance and maximizing impact. This responsiveness is crucial in maintaining a competitive edge in today's fast-paced market.
By integrating predictive analytics with existing marketing tools, businesses can create a seamless and efficient workflow that not only predicts market trends but also measures the return on investment (ROI) with greater accuracy.
The Adtech industry, in particular, has seen a significant transformation with the advent of predictive analytics. Real-time bidding, ad placement, and predictive campaign optimization are now conducted with a level of precision previously unattainable. This has led to more effective cross-channel advertising and a deeper understanding of audience segmentation.
Navigating Big Data: Challenges and Opportunities
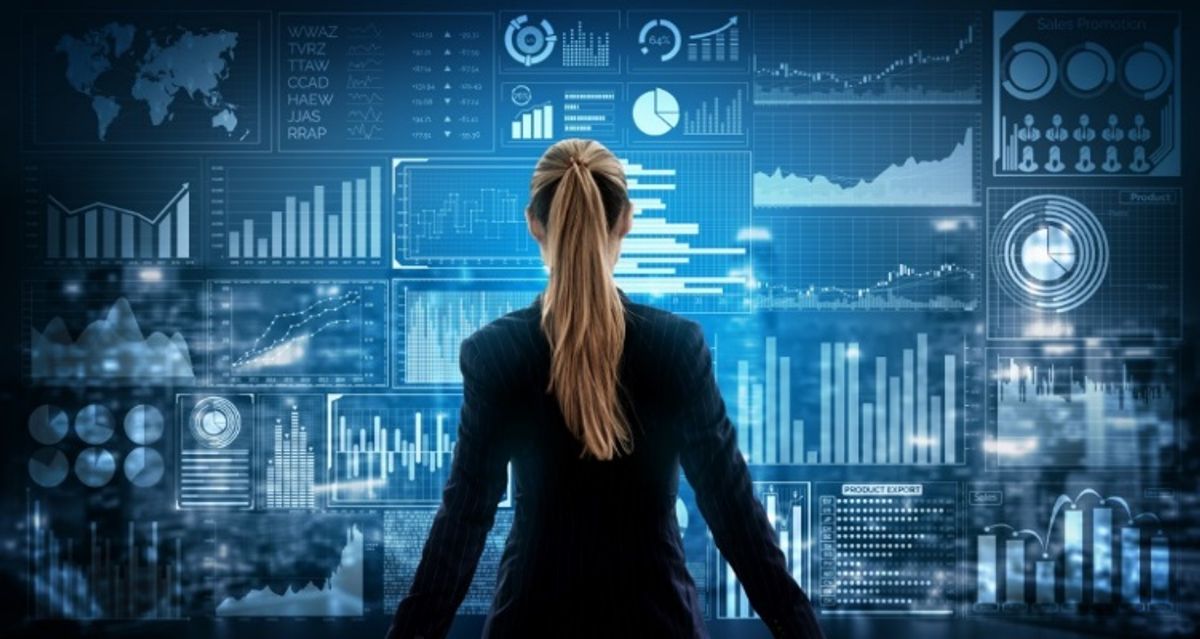
Handling Vast and Unstructured Data
In the era of big data, businesses are inundated with vast amounts of unstructured data. Navigating this data to extract meaningful insights is a formidable challenge that requires sophisticated tools and strategies. Open-source tools like Hadoop and NoSQL have emerged as pivotal in managing and processing these colossal datasets, offering scalability and agility to businesses.
The integration of unstructured data with traditional business analytics can reveal hidden issues and opportunities that might otherwise remain obscured.
Ensuring data quality is paramount; without it, even the most advanced analytics can lead to misguided decisions. Practices such as standardization, validation, and de-duplication are essential to maintain the integrity of data. Here's a succinct overview of key data management practices:
- Standardization: Ensuring uniform formats across data sets.
- Validation: Checking for accuracy and consistency in data.
- De-duplication: Removing duplicate entries to avoid redundancy.
By adopting a robust framework for data management, businesses can lay a foundation for deep insights, fostering synergies between data scientists and business intelligence professionals.
Synergies Between Data Scientists and BI Professionals
The collaboration between data scientists and business intelligence (BI) professionals is pivotal in sculpting a data-driven landscape within organizations. Data scientists provide advanced analytical capabilities, delving into predictive modeling and machine learning, while BI professionals focus on data extraction, reporting, and visualization to support decision-making processes.
- Data scientists explore and model data to forecast future outcomes and drive strategic planning.
- BI professionals use data warehousing and integration to ensure data consistency and reliability.
By fostering synergies between these roles, businesses amplify their ability to harness data for strategic advantage.
Adopting a culture of data-driven decision-making is essential. It involves promoting the use of BI tools across the organization and providing training to interpret and apply data effectively. This cultural shift empowers teams to leverage insights for informed decisions, maximizing the benefits of BI.
Big Data Analytics in Retail and Entertainment
In the competitive landscape of retail and entertainment, big data analytics stands as a transformative force. By analyzing intricate customer purchasing patterns and preferences, businesses like Walmart have optimized their inventory management to reflect real-world demand, setting optimal price points to maximize profits.
Netflix, with its vast subscriber base, exemplifies the power of data analytics in entertainment, tailoring content to viewer preferences and driving engagement.
- For physical stores, operational optimization and enhanced customer experiences are now within reach through actionable intelligence derived from customer data.
- Retailers are empowered to make evidence-based decisions, leading to increased customer satisfaction and profitability.
The fusion of large datasets with statistical analysis is revolutionizing decision-making, reshaping everything from healthcare initiatives to customer strategies in retail and entertainment.
The Convergence of AI and Analytics in Business Operations
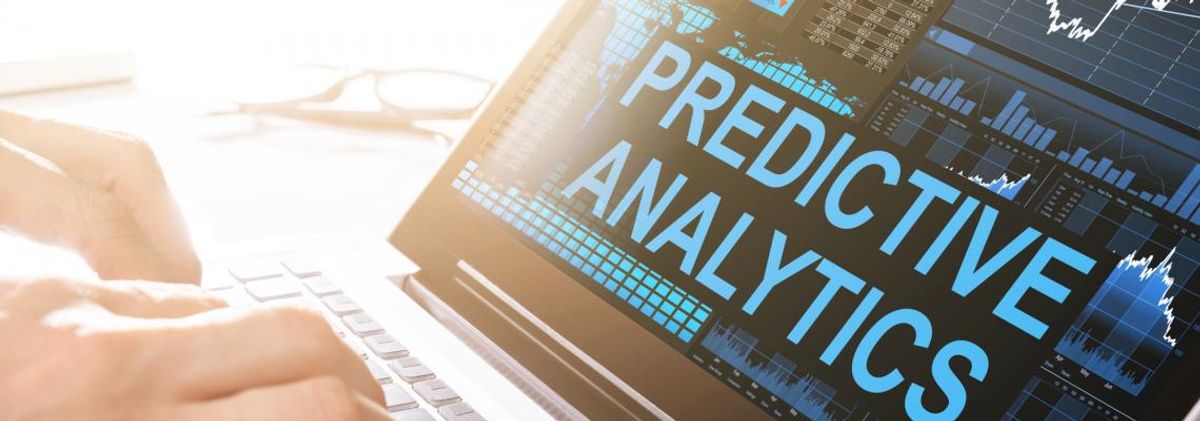
Automating Data Analysis Processes
The advent of artificial intelligence (AI) has revolutionized the way businesses approach data analysis. AI automates exploratory data analysis, enabling analysts to draw meaningful conclusions and inform decision-making more efficiently. This automation extends to generating predictive models, which are essential for forecasting and strategic planning.
One of the most anticipated advancements lies in the realm of automation, paired with diagnostic analytics. Automation streamlines data collection and analysis processes, allowing advanced systems to automatically analyze and present actionable insights. This not only reduces the margin of error but also significantly boosts efficiency.
By automating the mundane and repetitive tasks involved in data analysis, organizations can focus their human resources on more strategic and creative tasks.
Here's a brief overview of how automation impacts various stages of data analysis:
- Data Integration: Establishing strong connections between analytics platforms and existing tools.
- Data Analysis: Leveraging advanced analytics tools like data mining and machine learning to uncover patterns and insights.
- Data Visualization: Transforming complex data sets into understandable and actionable visual representations.
Navigating through workflow automation tools can streamline data processing, model deployment, and campaign execution. This not only accelerates time-to-insight but also frees up valuable resources for strategic initiatives.
AI-Driven Customer Insights
The advent of artificial intelligence (AI) in the realm of customer insights has revolutionized the way businesses understand and interact with their clientele. Integrating AI insights into business strategy involves more than just data analysis; it requires a cultural shift towards data-driven decision-making. This transformation is pivotal for companies looking to stay ahead in a competitive landscape.
AI-driven analytics offer a comprehensive view of the customer journey, highlighting not only future buying behaviors but also identifying potential disruptions. By leveraging these insights, businesses can proactively smooth out the customer experience, leading to enhanced engagement and profitability.
The synergy between AI and marketing strategies enables a level of personalization that elevates the customer journey from a mere transaction to a tailored, memorable experience.
To effectively harness the power of AI-driven insights, consider the following steps:
- Data Integration: Centralize data from various sources such as social interactions, purchase histories, and customer service engagements.
- Behavioral Analysis: Utilize machine learning to analyze past behaviors and forecast future ones.
- Segmentation: Form dynamic customer segments based on predictive behaviors.
- Personalization: Apply insights to target customers precisely, with messages that resonate on an individual level.
Case Studies: AI Transforming Industries
The advent of AI-powered predictive analytics has been a game-changer for industries across the board. Retailers, for instance, have seen a revolution in their business strategies, harnessing AI to extract deep insights from complex data sources. This has enabled them to not only enhance customer experiences but also to manage inventory and develop dynamic pricing with greater efficiency.
- AI-driven analytics optimize supply chains and maximize sales through strategic shelf arrangements.
- Personalized product recommendations are tailored to individual customer preferences, thanks to AI's interpretive capabilities.
The transformative power of AI extends beyond mere data analysis; it is reshaping entire business models, offering a competitive edge through personalized insights and cost-effective solutions.
Despite the clear benefits, some sectors have been hesitant to fully leverage AI, leaving a wealth of opportunities for innovation and real-time decision-making untapped. The challenge for these industries is to adapt quickly, integrating AI not just as a generative tool but as a strategic ally for precision and context-specific insights.
Building a Data-Centric Organizational Culture
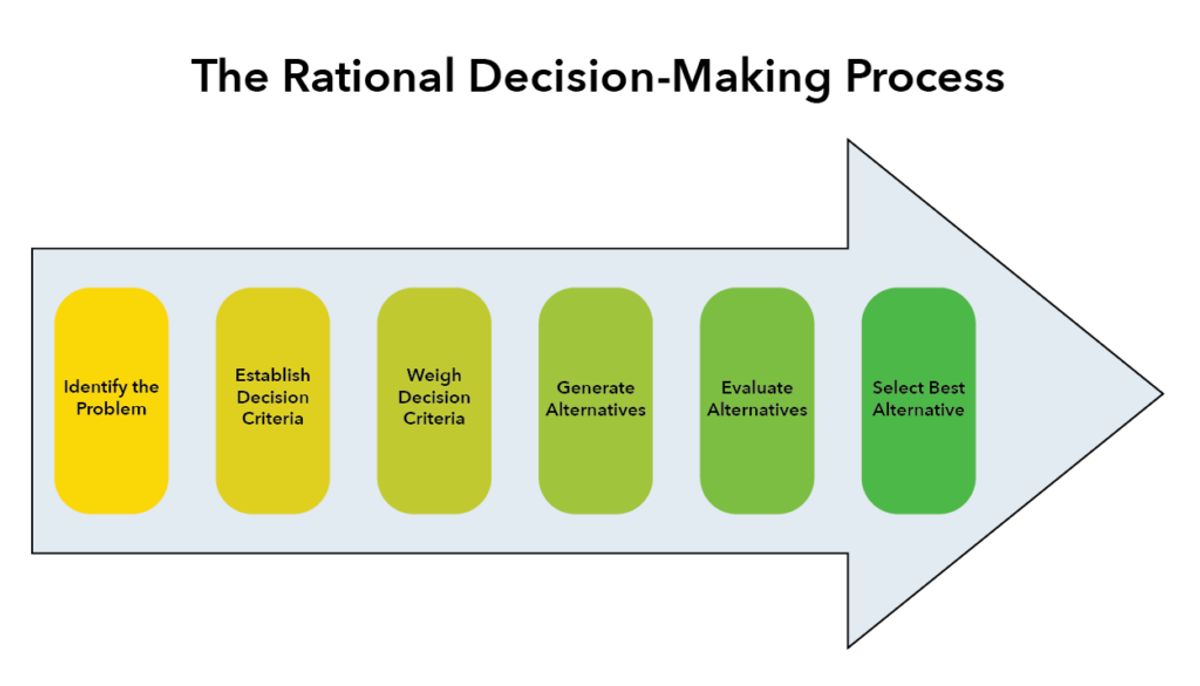
Fostering Data Literacy Among Employees
In the age of big data, data literacy is a crucial skill for all employees, not just data professionals. Fostering a culture of data literacy within an organization empowers every team member to make informed decisions. By providing training and resources, businesses can ensure that their employees are equipped to interpret and apply data effectively.
A culture of data-driven decision making is essential for leveraging the full potential of business intelligence (BI). It encourages objectivity and fairness in performance evaluations, helping to mitigate unconscious biases.
To cultivate this culture, consider the following steps:
- Encourage a culture of humility and curiosity.
- Promote inclusivity and belonging within teams.
- Leverage visual analytics to make complex data accessible.
- Incorporate analytics into performance evaluations for transparency.
By adopting these practices, organizations can create an environment where data is not only valued but is a cornerstone of the decision-making process.
The Role of Data Engineers in Decision-Making
In the realm of data-driven decision-making, data engineers play a pivotal role. They are the architects who construct the foundational frameworks that enable businesses to harness the power of data analytics. By transforming raw data into a structured and accessible format, data engineers ensure that actionable insights can be extracted efficiently.
Data quality is paramount, and it is the data engineer who lays the groundwork for maintaining its integrity. They implement systems that cleanse and validate data, ensuring that the analytics performed are based on accurate and reliable information.
- Design and maintenance of data systems
- Data cleansing and validation
- Development of data pipelines
- Integration of new data sources
Data engineers are instrumental in bridging the gap between raw data and actionable insights, making them an indispensable asset in the analytics process.
The collaboration between data engineers and analysts is essential for turning complex data sets into strategic business moves. With their technical expertise, data engineers empower organizations to navigate the vast seas of digital information and emerge with clear, data-driven directives.
Creating a Framework for Data-Driven Ethics
In the era of big data, establishing a framework for data-driven ethics is not just a regulatory necessity but a strategic imperative. Businesses must navigate the delicate balance between leveraging data for competitive advantage and respecting the privacy and rights of individuals. This involves clear policies on data usage, storage, and sharing, as well as transparency with stakeholders about how data informs business decisions.
To ensure ethical practices are embedded in the organizational culture, businesses should:
- Develop comprehensive data governance guidelines.
- Conduct regular ethics training for all employees handling data.
- Establish clear accountability for data breaches and misuse.
By prioritizing ethical considerations, companies can build trust with their customers and avoid the pitfalls of data misuse, which can lead to severe reputational damage.
The integration of prescriptive analytics and AI-driven data analytics is revolutionizing decision-making. Educating employees on the value of data and implementing ethical data practices are crucial for harnessing the transformative power of AI analytics for deeper insights.
The Future of Predictive Analytics in Business Innovation
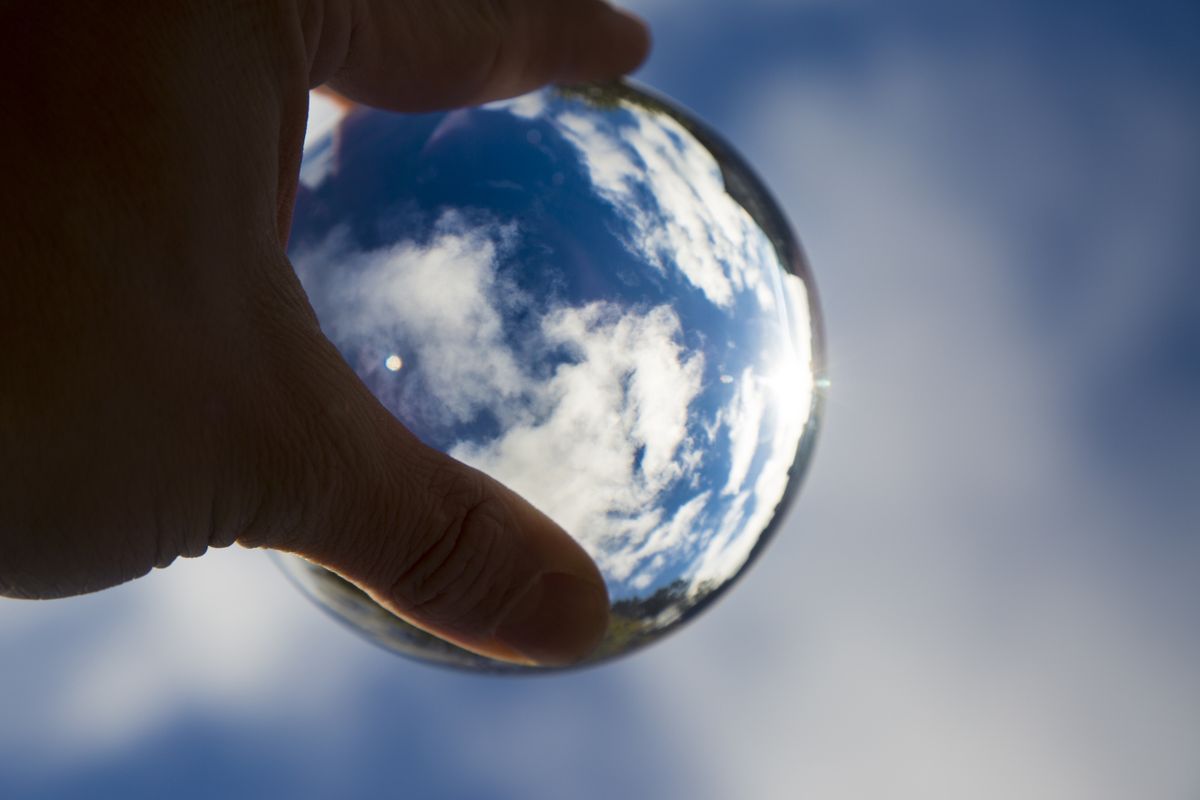
Emerging Trends in Data Analytics
The landscape of business analytics is continuously evolving, with 2024 poised to introduce several transformative trends. Augmented analytics is at the forefront, enhancing data processing and interpretation through the use of AI and machine learning. This trend is paving the way for more sophisticated and accessible insights.
Real-time analytics is another trend gaining momentum, allowing businesses to make decisions with unprecedented speed and agility. The ability to analyze data as it's generated provides a competitive edge in rapidly changing markets.
Data governance and privacy continue to be pivotal, as organizations navigate the complexities of regulatory compliance and ethical considerations in data usage.
Data democratization marks a significant shift towards empowering a broader range of users with access to analytics tools and insights. This trend is crucial for fostering a data-driven culture within organizations.
Here's a snapshot of the key trends:
- Augmented Analytics
- Real-Time Analytics
- Data Governance and Privacy
- Data Democratization
Predictive Analytics and Product Development
In the realm of product development, predictive analytics serves as a crystal ball, offering foresight into customer needs and market trends. By leveraging statistical modeling and machine learning, businesses can not only predict purchase intent but also innovate proactively, staying ahead of the curve.
Predictive analytics transforms the product lifecycle by enabling companies to anticipate demand, optimize inventory, and reduce the risk of obsolescence.
The integration of predictive analytics into product development can significantly enhance ROI by:
- Identifying potential market gaps and opportunities for innovation
- Tailoring products to meet evolving customer preferences
- Streamlining the supply chain to meet forecasted demand
By harnessing the power of data, businesses can not just meet, but exceed customer expectations, ensuring their offerings are always aligned with the market's pulse.
Anticipating Market Shifts with Data
In the ever-evolving landscape of business, anticipating market shifts has become a cornerstone of strategic planning. Predictive analytics serves as a crystal ball, offering glimpses into future trends and consumer behaviors. By harnessing this power, businesses can pivot before the market does, staying one step ahead of the competition.
Real-time data is the lifeblood of predictive analytics. It allows companies to adjust their strategies on the fly, ensuring that they remain relevant in a market where consumer preferences can shift overnight. The following list outlines the key benefits of using predictive analytics to anticipate market shifts:
- Proactive adaptation to changing market conditions
- Identification of emerging consumer trends
- Optimization of inventory and supply chain management
- Enhanced ability to forecast sales and demand
With predictive analytics, businesses are not just reacting to changes; they are preparing for them, crafting strategies that are both resilient and dynamic.
Ensuring the quality of data is paramount; without it, the insights gleaned from analytics are compromised. A robust data management system is the foundation upon which accurate predictions are built. It's not just about having data; it's about having data that is clean, structured, and validated.
As businesses continue to navigate the ever-evolving landscape of technology, the role of predictive analytics becomes increasingly critical in driving innovation. At OptimizDBA, we understand the urgency to stay ahead of the curve. Our proprietary methodologies and unparalleled experience in database optimization ensure that your company can leverage data at speeds that outpace the competition. Don't let your business fall behind; visit our website to discover how we can transform your data-driven strategies and propel your business forward.
Conclusion
In conclusion, predictive analytics stands as a transformative force in the realm of business decision-making. Throughout this article, we have seen how leveraging data not only sharpens competitive edges but becomes an indispensable asset in a data-driven world. From enhancing marketing strategies to optimizing operations and customer journeys, the integration of predictive analytics into business processes enables organizations to anticipate market trends, adapt to consumer needs, and make strategic decisions with greater confidence. The case studies and insights presented underscore the profound impact that data analytics has on profitability and efficiency. As businesses continue to navigate the complexities of the digital landscape, the ability to translate data into actionable insights will remain a critical component of success. Embracing this data-centric approach will empower businesses to not only survive but thrive in an increasingly competitive and ever-evolving marketplace.
Frequently Asked Questions
What is predictive analytics and how does it impact business decision-making?
Predictive analytics involves using historical data, statistical algorithms, and machine learning techniques to identify the likelihood of future outcomes. It helps businesses anticipate trends, understand customer behavior, and make informed strategic decisions.
How can predictive analytics give a competitive advantage to businesses?
By forecasting future trends and customer behaviors, predictive analytics allows businesses to stay ahead of the curve, optimize operations, tailor marketing efforts, and ultimately drive profitability.
What role does data quality play in data-driven decision-making?
High-quality data is essential for accurate analysis and reliable predictions. It ensures that the insights and forecasts derived from predictive analytics are trustworthy and actionable.
How is machine learning transforming business forecasting?
Machine learning algorithms can uncover complex patterns in data that are not apparent to human analysts. This capability enables more precise and nuanced forecasting in various business functions.
In what ways can predictive analytics optimize marketing strategies?
Predictive analytics can enhance customer journey analysis, improve targeting and personalization, and help marketers allocate resources more effectively to maximize ROI.
What are the challenges of navigating big data in predictive analytics?
Challenges include managing the volume, velocity, and variety of big data, ensuring data quality and security, and the need for specialized skills to analyze and interpret the data.
How is AI converging with analytics in business operations?
AI is automating data analysis processes, providing deeper customer insights, and enabling real-time decision-making, thereby transforming various industries and their operational efficiencies.
What trends are shaping the future of predictive analytics in business?
Emerging trends include the integration of AI and machine learning, the increasing importance of data ethics, and the continuous development of tools and technologies that enhance data processing and analysis.