Unleashing the Power of Predictive Analytics
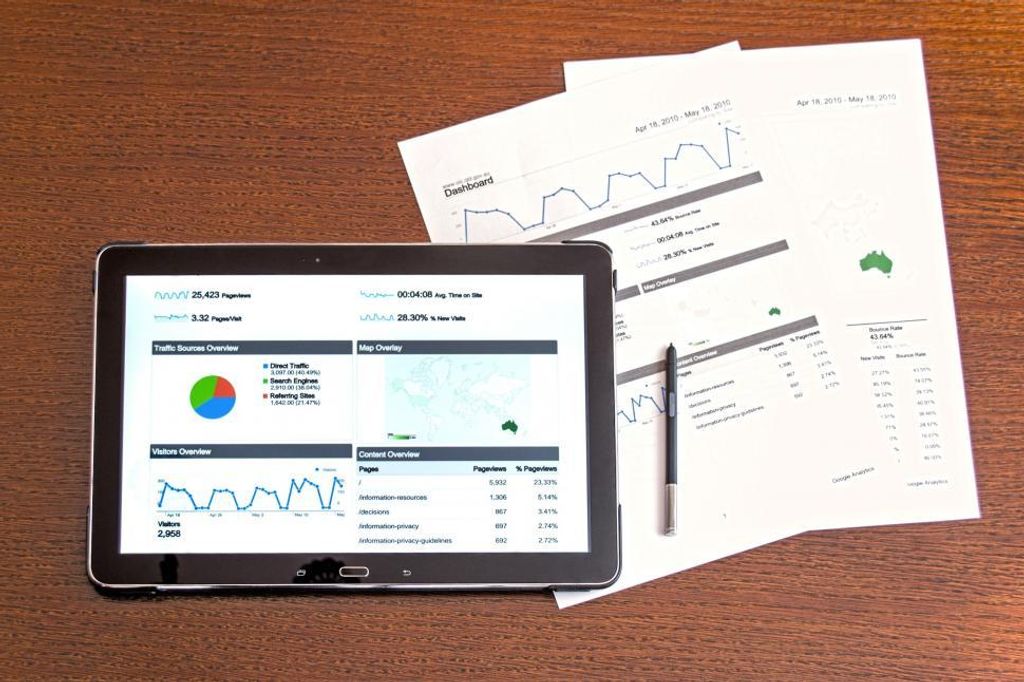
Utilizing Historical Data Patterns
The art of leveraging historical data is foundational in predictive analytics. By examining past performance, businesses can discern patterns and trends that are instrumental in forecasting future outcomes. This process often begins with descriptive analytics, which involves a thorough analysis of historical data to understand past trends and performance.
Historical data analysis is not just about looking back; it's a stepping stone towards predictive insights that drive business strategy forward.
To effectively utilize historical data patterns, several steps are typically followed:
- Querying: Gathering targeted information by querying databases.
- Descriptive analytics: Understanding past trends and patterns.
- Statistical analysis: Identifying statistical patterns and insights.
- Data visualization: Enhancing comprehension through visual representations.
These steps ensure that the insights derived are not only accurate but also actionable, enabling businesses to make informed decisions. The table below succinctly presents the key components of utilizing historical data patterns:
Component | Description | Benefit |
---|---|---|
Querying | Access to targeted information | Focused analysis |
Descriptive Analytics | Examining historical data | Understanding of past trends |
Statistical Analysis | Applying statistical techniques | Identification of insights |
Data Visualization | Visual representations of data | Improved data comprehension |
Implementing Machine Learning Algorithms
The integration of machine learning algorithms into business analytics marks a significant leap towards actionable insights. Advanced machine learning algorithms are pivotal in adapting to evolving patterns and trends, which enhances the accuracy and relevance of insights over time.
By automating the analysis of large, complex data sets, these algorithms generate recommendations for specific courses of action, optimizing decision-making in real-time.
To successfully implement machine learning in your business analytics, consider the following steps:
- Define specific goals for your AI implementation that are measurable and achievable.
- Identify and organize the necessary data sources to train your AI models.
- Choose your AI tools, exploring both free and paid platforms to find the best fit for your needs.
While the benefits of adopting such advanced analytics are substantial, including increased agility and improved decision accuracy, businesses must also navigate challenges related to data quality and consistency. Ensuring the accuracy, relevance, and consistency of your data is imperative for generating reliable insights.
Driving Performance and Growth
In the realm of business analytics, driving performance and growth is paramount. By harnessing the power of data, companies can optimize operational performance, enhance customer experience, and uncover hidden patterns and trends that lead to new business opportunities.
- Make data-driven decisions
- Identify new business opportunities
- Optimize operational performance
- Enhance customer experience
Cloud computing offers cost savings, scalability, and flexibility for businesses. It enhances collaboration, improves data security, and optimizes operations for business success.
As businesses manage large volumes of data, ensuring data security and privacy becomes crucial. Integrating disparate data sources and processing data in real-time are challenges that require acquiring the necessary technical expertise. The journey toward data-driven decision making is a transformative one, with analytics at its core, shaping business strategies for a competitive and adaptable future.
Building a Data-Driven Culture
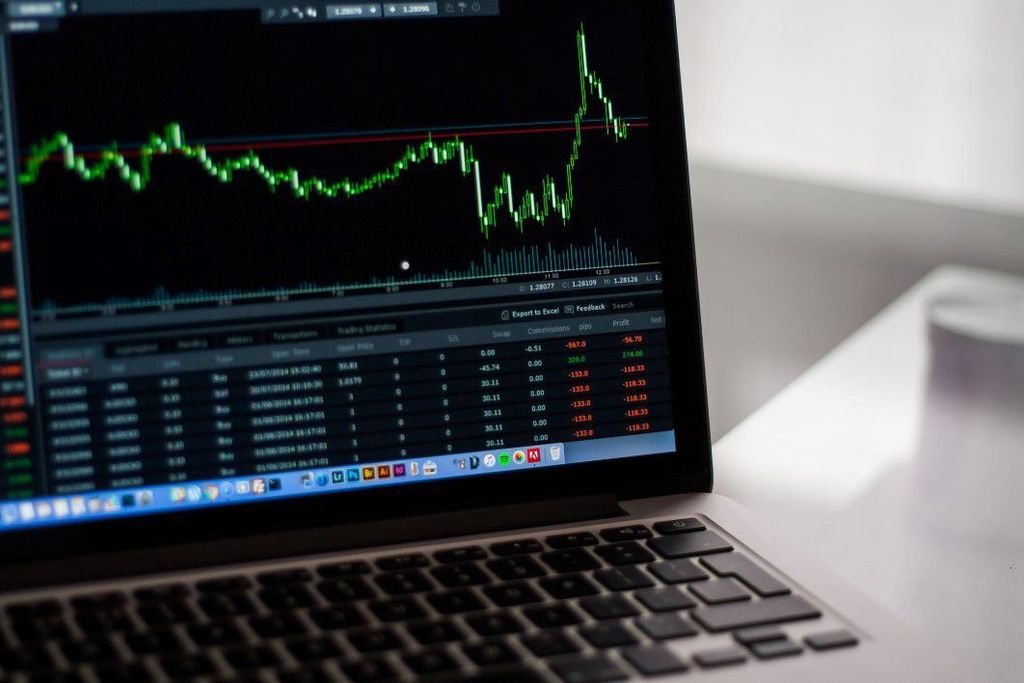
Importance of Data for Insights
In the realm of modern business, data is the cornerstone of insight generation. It fuels the predictive engines that drive strategic decision-making and operational improvements. By analyzing consumer demographics and behaviors, businesses can tailor their offerings to meet the precise needs of their target audience, ensuring they reach the right people, at the right place, at the right time.
Data quality is paramount; without it, insights may be misleading. To ensure the integrity of data insights, consider the following points:
- Data Quality and Consistency: High-quality data sets are crucial for accurate analytics.
- Balancing Data and Intuition: Combine data insights with human intuition for well-rounded decisions.
- Integration with Existing Systems: Seamless integration with current systems enhances data utility.
Embracing a data-driven approach empowers organizations to not only react to market conditions but to anticipate and shape them. This proactive stance is essential for maintaining a competitive edge in today's fast-paced business environment.
Steps to Implementing Data-Driven Culture
Establishing a data-driven culture is a multifaceted process that requires careful planning and execution. The journey begins with assessing your current data maturity level to understand where your organization stands and what steps are needed to progress.
- Step 1: Define clear objectives and metrics to guide your data initiatives.
- Step 2: Secure leadership buy-in to ensure organizational alignment and support.
- Step 3: Invest in the right technology and tools to facilitate data collection and analysis.
- Step 4: Develop robust data management processes for data quality and consistency.
- Step 5: Balance data insights with human intuition and creativity.
It is crucial to communicate the significance of data intelligence initiatives and involve employees at every stage to overcome cultural resistance and foster acceptance.
By following these steps, organizations can lay a solid foundation for a data-driven decision-making process that harnesses the full potential of their data for a competitive edge.
Benefits and Challenges of Data Analytics Adoption
The journey towards a data-driven enterprise is marked by significant milestones of success and hurdles that require strategic navigation. Big data fuels analytical models, enabling informed decision-making and business growth. However, the path is not without its obstacles.
Challenges in data analytics necessitate innovative solutions, such as robust data governance frameworks and the deployment of advanced analytics tools. These solutions are critical in ensuring data quality and consistency, which are the bedrock of reliable analytics.
Embracing the benefits while overcoming the challenges is a balancing act that demands attention to detail and a commitment to continuous improvement.
The following list outlines some key benefits and challenges of data analytics adoption:
- Benefits:
- Increased agility in decision-making
- Improved decision accuracy
- Enhanced operational efficiency
- Challenges:
- Ensuring data quality and consistency
- Developing data literacy and analytical skills
- Incorporating data insights into decision-making processes
- Conducting regular data analysis to stay informed
By addressing these challenges head-on, organizations can fully leverage the benefits and transform their operations through data-driven insights.
Enhancing Business Intelligence with Advanced Analytics
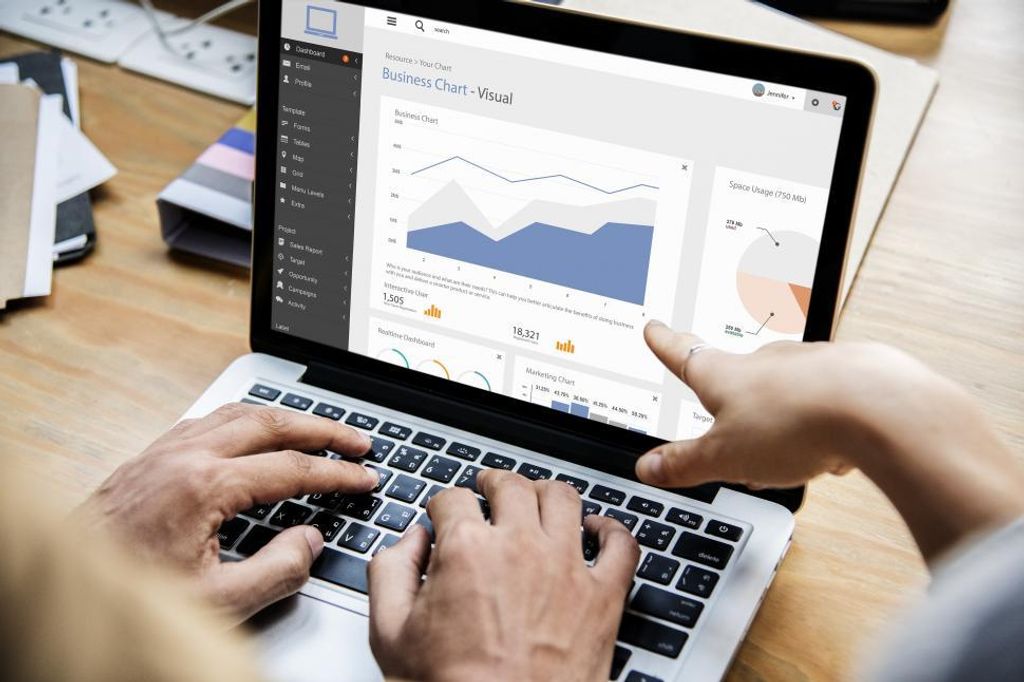
Differentiating BI and Business Analytics
Understanding the distinction between Business Intelligence (BI) and Business Analytics is crucial for leveraging the full potential of data in decision-making. BI is primarily concerned with what happened in the past and what is happening now. It uses data mining, reporting, and descriptive analytics to provide a comprehensive view of an organization's data, which aids in driving change and adapting to market conditions. In contrast, Business Analytics is forward-looking; it applies insights from data analysis to drive action and optimize future business performance.
To illustrate the differences more clearly, consider the following table:
Aspect | Business Intelligence (BI) | Business Analytics |
---|---|---|
Focus | Past and present data | Future-oriented insights |
Primary Goal | Inform decision-making | Drive action and optimization |
Key Techniques | Data mining, Reporting | Predictive, Prescriptive |
Outcome | Understanding of trends | Strategic business decisions |
While both BI and Business Analytics are integral to a data-driven organization, they serve different purposes. BI helps in understanding where you have been, while Business Analytics informs where you are going.
It's important to recognize that these two fields do not operate in isolation but rather complement each other. Together, they empower organizations to make informed decisions, identify areas for improvement, and respond to market dynamics effectively.
Visualizing BI Processes
Visualizing BI processes is a critical step in bridging the gap between raw data and actionable insights. Interactive visual analysis allows stakeholders to explore data through visual interfaces, leading to the discovery of valuable insights. The process begins with data preparation, where raw data is cleaned, transformed, and organized to become analysis-ready.
To illustrate the flow of BI processes, consider the following table:
BI Process | Description | Key Outcome |
---|---|---|
Data Mining | Extracting patterns and trends from large datasets | Identification of valuable insights |
Reporting | Preparing structured reports for decision-making | Clear information dissemination |
Descriptive Analytics | Examining historical data for past performance | Understanding of past trends |
Querying | Accessing specific datasets for analysis | Quick retrieval of needed information |
By leveraging these BI methods, organizations can harness data for customer retention, marketing strategy, and operational efficiency. Segmentation for targeted marketing becomes more precise, and a competitive edge is gained through data analytics.
Embracing BI processes is essential for a comprehensive view of the organization, enabling informed decision-making and identifying areas for improvement.
Leveraging Data Insights for Action
In the realm of business intelligence, the true value of analytics is realized when insights are transformed into strategic action. Data insights serve as a compass, guiding companies through the complexities of the market and informing critical decisions. To effectively leverage these insights, businesses must establish clear processes that translate data findings into operational improvements and innovative strategies.
Actionable insights are not just about understanding what has happened or what is happening; they are about predicting what could happen and preparing for it. Here's a simple framework to turn insights into action:
- Identify key performance indicators (KPIs) relevant to business objectives.
- Analyze data patterns and trends against these KPIs.
- Develop hypotheses for potential outcomes based on insights.
- Test these hypotheses through controlled experiments or pilot programs.
- Implement successful strategies at scale across the organization.
By embedding data insights into the decision-making process, businesses can ensure a proactive stance towards market dynamics, driving growth and maintaining a competitive edge.
It is crucial for organizations to not only gather and analyze data but also to create an environment where insights are shared and acted upon. This requires a culture that values data literacy and empowers employees to make data-informed decisions. The journey from data to action is iterative and demands continuous learning and adaptation.
AI-Powered Business Analytics
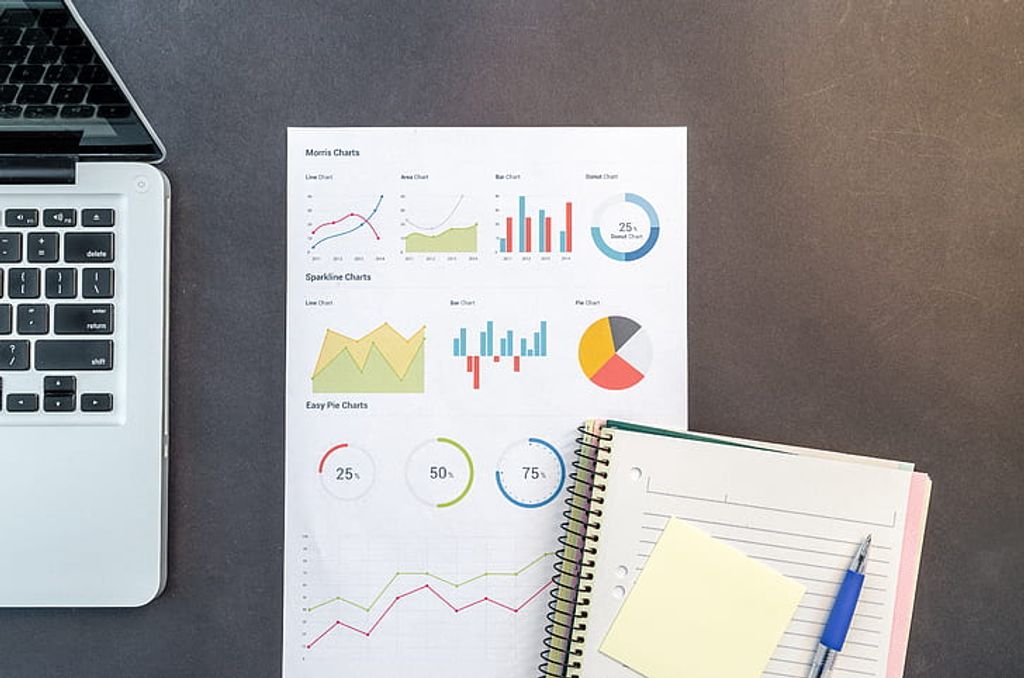
AI Techniques for Advanced Analytics
The advent of Artificial Intelligence (AI) has revolutionized the way businesses approach analytics. By integrating AI techniques into their analytics frameworks, companies can now process and interpret vast amounts of data with unprecedented speed and accuracy. AI enables the transformation of raw data into predictive insights, facilitating proactive decision-making and strategic planning.
Predictive analytics is a cornerstone of AI in business, leveraging historical data and machine learning to forecast future trends and behaviors. This allows businesses to make timely adjustments to their strategies, mitigate risks, and capitalize on emerging opportunities. Other AI techniques such as natural language processing and deep learning further enhance the analytical capabilities, providing a more nuanced understanding of data patterns.
AI-powered analytics offers a competitive edge by automating complex processes and generating insights that are beyond the reach of traditional analytics methods.
The table below outlines some of the key AI techniques used in advanced analytics:
Technique | Description |
---|---|
Predictive Analytics | Utilizes historical data to predict future outcomes. |
Machine Learning | Employs algorithms to learn from data and improve over time. |
Natural Language Processing | Analyzes human language to extract meaningful information. |
Deep Learning | Uses neural networks to model and understand complex patterns. |
Benefits of AI in Business Analytics
The integration of AI into business analytics has revolutionized the way companies interpret data and make decisions. AI enhances the speed and accuracy of data analysis, allowing businesses to respond more quickly to market changes and customer needs. With AI, complex data sets are processed and analyzed with a level of depth and understanding that was previously unattainable.
- Improved Decision-Making: AI algorithms can identify patterns and trends that humans might overlook, leading to more informed and strategic decisions.
- Increased Efficiency: Automation of routine data analysis tasks frees up valuable time for employees to focus on more strategic activities.
- Personalization: AI enables businesses to tailor experiences and products to individual customer preferences, driving engagement and loyalty.
By leveraging AI, businesses can gain a significant competitive advantage, as they are equipped to predict future trends and customer behaviors with a high degree of precision. This proactive approach to analytics can be a game-changer in any industry.
Implementing AI in Business Analytics
Implementing AI in your business analytics can be a transformative step, offering a competitive edge in today's data-driven landscape. Embrace the future by understanding the benefits, challenges, and practical steps to integrate AI into your analytics strategy.
To begin, define your AI mission by identifying your most pressing business challenges and areas where AI can provide valuable insights. For instance, improving customer churn prediction or optimizing marketing campaigns. Set SMART goals to ensure your AI initiatives are focused and measurable.
Here's a practical guide to get you started:
- Phase 1: Define Your AI Mission
- Phase 2: Assess Your Data Infrastructure
- Phase 3: Select the Right AI Tools
- Phase 4: Train Your Team
- Phase 5: Deploy AI Solutions
- Phase 6: Monitor and Refine
AI is a powerful tool, but its success hinges on continuous learning, effective collaboration, and responsible deployment. Start your AI-powered business analytics journey today and unlock the full potential of your data.
Remember, AI is not just for large enterprises. Businesses of all sizes can leverage AI to enhance their analytics capabilities and achieve remarkable results. Take the time to explore AI tools tailored to your industry and budget.
Transforming Business Strategy with Analytics
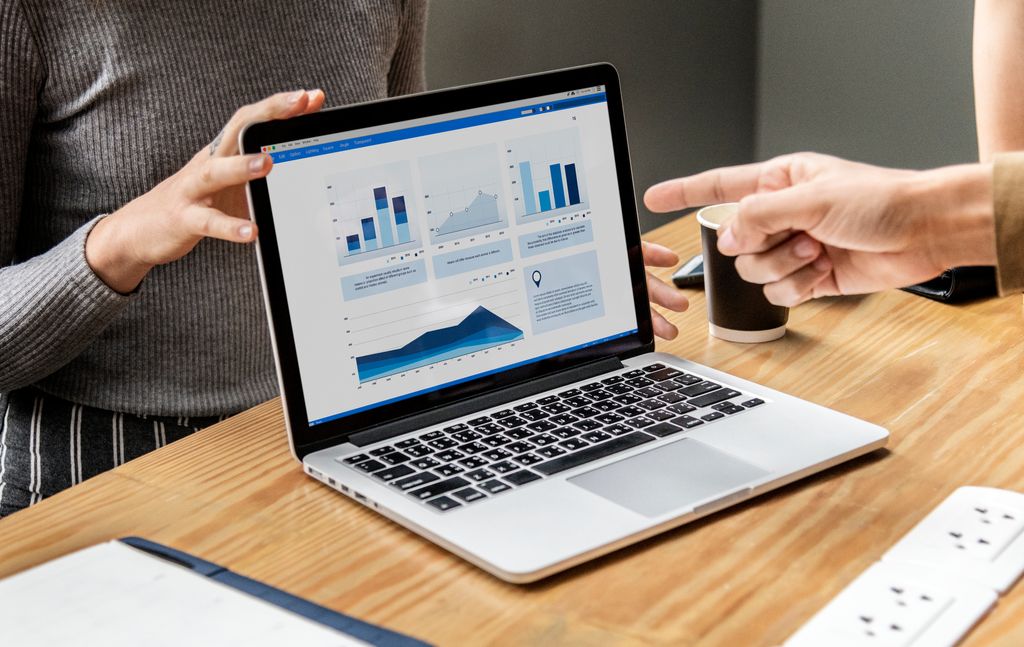
Harnessing Data for Strategic Decisions
In the realm of modern business, data-driven decision making stands as a cornerstone of strategic planning. By tapping into the wealth of information available, companies can uncover insights that lead to more informed and effective strategies. The agility gained from this approach allows businesses to not only anticipate future trends but also respond rapidly to changes in the market.
The ability to convert raw data into actionable insights is crucial for optimizing strategies and achieving sustained growth.
To fully capitalize on the power of data, organizations must focus on key areas:
- Identifying relevant data sources
- Developing robust analytical models
- Cultivating a culture that values data literacy
Each of these steps is essential for transforming data into a strategic asset that drives performance and maintains a competitive edge.
Forecasting Trends and Improving Efficiency
In the realm of business strategy, the ability to forecast trends and enhance efficiency hinges on the sophisticated use of analytics. Predictive analytics is a cornerstone in this domain, leveraging historical data and machine learning to anticipate future events. This foresight enables businesses to align their resources with projected demands, ensuring a more efficient operation.
By integrating data and real-time trends, AI not only refines sales forecasting but also streamlines inventory management. The result is a significant reduction in stockouts and unnecessary costs, exemplifying the transformative impact of analytics on efficiency.
Prescriptive analytics complements predictive efforts by not just forecasting outcomes, but also recommending actionable steps. Here's how these analytics types interact:
- Predictive analytics forecasts future events based on historical data.
- Prescriptive analytics suggests actions to influence desired outcomes, integrating business rules and algorithms.
Together, these analytical approaches empower businesses to make timely adjustments, mitigate risks, and seize emerging opportunities. The future of enterprise data intelligence is bright, with trends pointing towards more automated and reliable technologies that enhance semantic understanding and decision-making processes.
Adapting to Market Conditions
In the dynamic landscape of modern business, the ability to swiftly adapt to market conditions is not just an advantage—it's a necessity. Real-time data access is the cornerstone of this adaptability, providing businesses with the agility to respond to evolving trends, customer behaviors, and competitive movements.
Predictive analytics plays a pivotal role in proactive decision-making. By analyzing historical data and employing statistical algorithms, businesses can forecast future events and trends, positioning themselves to preemptively address market shifts. The following table summarizes the key aspects of predictive analytics:
Aspect | Description |
---|---|
Historical Data | Foundation for identifying patterns and predicting outcomes. |
Statistical Models | Tools for analyzing data and forecasting trends. |
Machine Learning | Enables continuous improvement in prediction accuracy. |
Embracing advanced analytics allows for timely adjustments to strategies, mitigating risks, and capitalizing on opportunities. It's about transforming data into a strategic asset that informs every decision, ensuring that businesses are not just reacting to the market, but shaping it.
While the benefits of a data-driven approach are clear, such as increased agility and improved decision accuracy, challenges like ensuring data quality and consistency must be addressed. Developing a robust analytics framework is essential for businesses to thrive in an ever-changing market.
Driving Growth through Data Literacy
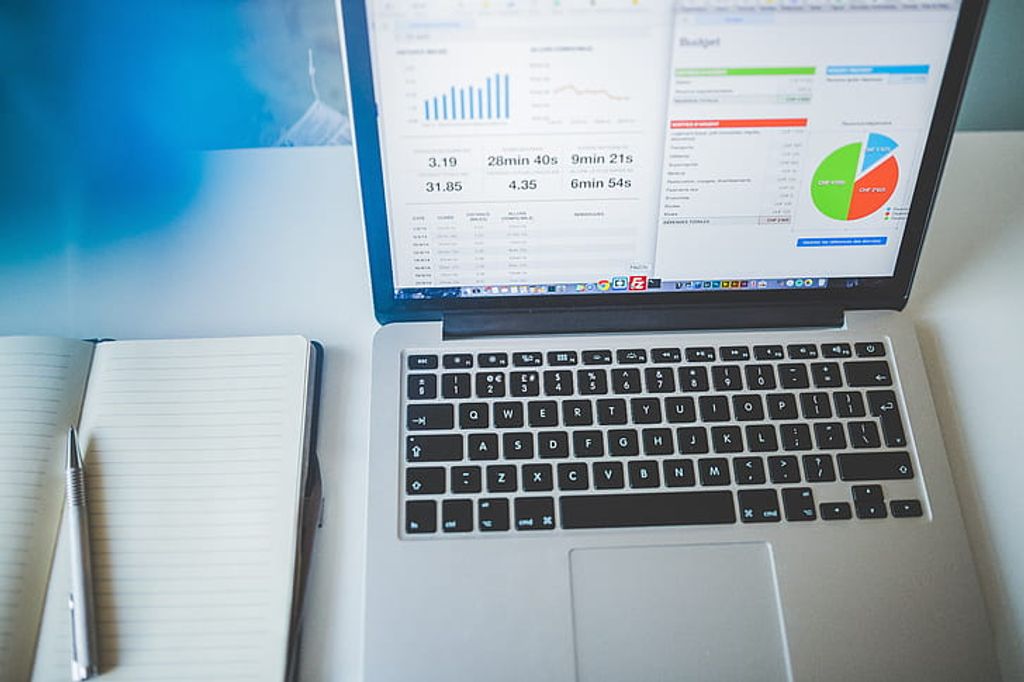
Developing Analytical Skills
In the era of big data, developing analytical skills across your team is not just an advantage; it's a necessity. These skills enable individuals to interpret complex data, recognize patterns, and make informed decisions. To foster these abilities, organizations must focus on structured training programs that cover both the theoretical and practical aspects of data analytics.
Data literacy is the cornerstone of a data-driven culture. It involves understanding what data is relevant, how to process it, and the implications of the insights derived. Here's a simple framework to begin enhancing analytical skills within your team:
- Foundation: Establish a baseline of knowledge with fundamental concepts of statistics and data analysis.
- Tools: Train on specific analytics tools and software that are widely used in the industry.
- Application: Engage in real-world projects to apply analytical concepts and tools.
- Evaluation: Regularly assess skills and knowledge to identify areas for improvement.
By investing in the development of analytical skills, organizations can ensure that their workforce is equipped to handle the challenges of a data-rich environment. This investment not only improves individual competencies but also contributes to the overall analytical maturity of the business.
Incorporating Data Insights in Decision-Making
In the realm of business, the integration of data insights into decision-making processes is not just a trend but a fundamental shift towards a more analytical approach. Data-driven decisions are the cornerstone of a modern, agile business strategy, enabling companies to respond with precision to the ever-changing market landscape.
To effectively incorporate data insights, businesses must first establish clear protocols for data analysis and interpretation. The following steps can serve as a guideline:
- Collect relevant data from various sources.
- Cleanse and organize the data to ensure accuracy.
- Analyze the data to uncover patterns and trends.
- Translate insights into actionable strategies.
- Implement decisions and monitor outcomes for continuous improvement.
By embedding data insights into the core of decision-making, businesses can enhance their agility and operational efficiency. This proactive stance allows for a more nuanced understanding of the business environment, leading to strategic actions that are both informed and impactful.
It is crucial to recognize that while data can significantly improve decision-making, it is not an infallible source. Decisions should be balanced with experience and intuition to navigate the complexities of the business world. The best BI tools simplify these data complexities, cater to all sizes of businesses, and ultimately enhance decision-making. Discovering the right BI tool tailored to your business needs is a step towards harnessing the full potential of data.
Conducting Regular Data Analysis
Regular data analysis is a cornerstone of a data-literate organization. It ensures that decision-making is grounded in facts and trends rather than intuition. Conducting analysis frequently allows businesses to stay agile, adapting to new information as it becomes available.
Data visualization and visual analysis are key components in this process. They transform complex data sets into understandable and actionable insights. For instance, visual representations such as charts and graphs can quickly convey the status of key performance indicators (KPIs).
By embedding regular data analysis into the workflow, organizations can foster a culture of continuous improvement and data-driven decision-making.
The following table summarizes some of the most effective business analysis techniques:
Technique | Description |
---|---|
Data Mining | Uncovering trends and patterns from large datasets |
Reporting | Preparing structured reports for decision-making |
Descriptive Analytics | Understanding past trends and performance |
Statistical Analysis | Exploring data relationships and making predictions |
Data Visualization | Presenting data visually for better understanding |
Adopting techniques such as Business Process Modeling (BPM) and MOST (Mission, Objectives, Strategies, Tactics) can further enhance the analytical framework, ensuring that the insights gained are aligned with the organization's strategic goals.
Conclusion
In conclusion, the journey of transforming data into actionable insights through robust business analytics is a critical endeavor for modern organizations. By leveraging advanced analytical techniques, cultivating a data-driven culture, and investing in team training, businesses can unlock the full potential of their data to drive strategic decision-making and sustainable growth. Embracing the power of data analytics is not just a trend but a necessity in today's competitive landscape. As businesses continue to evolve, the integration of data-driven analytics will be key to staying ahead of the curve and making informed, impactful decisions. The future of business analytics is bright, and those who embrace it will thrive in the data-driven era.
Frequently Asked Questions
What is the importance of data for insights in business analytics?
Data is crucial for generating meaningful insights in business analytics as it provides the foundation for understanding trends, patterns, and connections within the data sets.
How can businesses implement AI in business analytics?
Businesses can implement AI in business analytics by leveraging AI techniques such as machine learning algorithms and natural language processing to analyze data and generate actionable insights.
Why is developing data literacy important for driving growth through data analytics?
Developing data literacy is important as it equips individuals with the skills to interpret and leverage data insights effectively, enabling informed decision-making and driving growth.
What are the benefits of leveraging advanced analytics in business strategy?
Leveraging advanced analytics in business strategy enables organizations to forecast trends, improve efficiency, and adapt to market conditions swiftly, leading to strategic decision-making and enhanced agility.
How can businesses differentiate between Business Intelligence (BI) and Business Analytics?
Business Intelligence focuses on extracting insights from historical data for decision-making, while Business Analytics applies insights to drive action and optimize performance, emphasizing a more proactive approach.
What are the key steps to implementing a data-driven culture in an organization?
The key steps to implementing a data-driven culture include fostering data literacy, encouraging data-driven decision-making, investing in team training, and conducting regular data analysis.
How does predictive analytics contribute to driving performance and growth in business analytics?
Predictive analytics utilizes historical data patterns and machine learning algorithms to forecast future trends, identify opportunities, and optimize strategies, leading to improved performance and growth.
Why is it essential for businesses to adapt to market conditions through data-driven decision-making?
Adapting to market conditions through data-driven decision-making allows businesses to stay competitive, respond to changing trends, and capitalize on emerging opportunities, ensuring long-term success.